Mitigating Racial Bias in Health Care Algorithms: Improving Fairness in Access to Supportive Housing
Abstract
Algorithms for guiding health care decisions have come under increasing scrutiny for being unfair to certain racial and ethnic groups. The authors describe their multistep process, using data from 3,465 individuals, to reduce racial and ethnic bias in an algorithm developed to identify state Medicaid beneficiaries experiencing homelessness and chronic health needs who were eligible for coordinated health care and housing supports. Through an iterative process of adjusting inputs, reviewing outputs with diverse stakeholders, and performing quality assurance, the authors developed an algorithm that achieved racial and ethnic parity in the selection of eligible Medicaid beneficiaries.
HIGHLIGHTS
Many algorithms used in health care decisions have the potential to create or perpetuate health care disparities between people identifying as non-Hispanic White and persons of color.
By continually revising inputs and reviewing outputs of multiple iterations, the authors developed an algorithm that demonstrated racial and ethnic parity in selecting persons experiencing homelessness and chronic health problems who were eligible to receive coordinated health care and housing supports.
There is increasing recognition that many of the algorithms used in health care decision making, particularly decisions about who can access specialty programs, are subject to systematic errors in accurately classifying and selecting persons of color who are eligible for such programs, which can perpetuate and potentially amplify racial inequities in health outcomes (1). For example, recent research revealed that a widely used commercial algorithm designed to optimize referrals for high-risk care management programs incorrectly classified Black patients as having less need for care than equally ill White patients (2). Because the algorithm relied on health care costs as an indicator of patient health, it identified less than half of eligible Black patients, who, because of lower access to care, tended to have lower health care costs despite experiencing need equal to that of White patients. In response to rising concerns about algorithmic bias, in 2021 the U.S. Food and Drug Administration released an action plan and guidance for developing and deploying machine-learning approaches to advance health equity (3). Despite considerable effort and attention expended on addressing this issue in recent years, many questions remain about how best to define and measure algorithmic fairness and equity, given that many approaches are incompatible with one another (4, 5).
Stable and safe housing is increasingly seen as a critical determinant of health equity (6). However, shortages in affordable housing units are a primary driver of housing instability and homelessness, which disproportionately affect persons of color (7). In this column, we summarize the iterative process we undertook to identify and reduce racial and ethnic bias in an algorithm developed for a new statewide initiative to improve housing stability and health outcomes for eligible Medicaid members in Connecticut. More specifically, the state’s behavioral health administrative service organization (ASO) for Medicaid was charged with developing a process to identify eligible beneficiaries experiencing homelessness, as documented in the statewide Homeless Management Information System (HMIS), and having complex health conditions and needs. The premise was that these beneficiaries would improve their health and quality of life by receiving housing supports provided by a Medicaid- and state-funded adult housing program.
The Housing Support Program
The program was developed in 2019–2021 with the aim of promoting health and well-being by coordinating targeted health care and housing support services for Medicaid beneficiaries experiencing homelessness or housing insecurity. Connecticut has a shortage of approximately 89,000 affordable rental homes for extremely low-income households (https://www.ctinsider.com/politics/article/affordable-housing-shortage-ct-us-18531832.php). Building on past successes with supportive housing initiatives as well as a Money Follows the Person demonstration project, this program will focus on helping eligible individuals experiencing homelessness to find and stay in affordable housing and connecting them with appropriate services and supports. The program represents a partnership between multiple state agencies (e.g., the state Medicaid authority, the adult mental health and addiction authority, housing services, and the housing finance authority) and private partners (e.g., various housing advocacy and support organizations). The housing support program includes a person-centered recovery plan to assist individuals through coordinated care that addresses health care needs and through housing vouchers to promote stable housing with pretenancy supports, tenancy-sustaining supports, and nonmedical transportation. Medicaid members are assessed for eligibility if they have spent at least one night in a homeless shelter or otherwise meet the criteria stipulated by the U.S. Department of Housing and Urban Development for being homeless (category 1) or fleeing domestic violence (category 4). According to HMIS estimations, the homeless population in Connecticut is roughly 27% Hispanic, 30% White, and 30% Black or African American (https://cceh.org/equity-dashboard; we note that Hispanic ethnicity is no longer reported as a separate category).
Algorithm Development
To help identify the individuals most likely to benefit from the housing support program, the ASO was tasked with developing an algorithm that would achieve four objectives. First, it would comply with applicable laws and regulations about program eligibility criteria. Second, it would identify for outreach a sufficient number (i.e., volume) of eligible Medicaid beneficiaries to meet the target of enrolling up to 850 people to receive housing vouchers and coordinated health care over 5 years (i.e., about 170 beneficiaries per year). Third, it would not disproportionately select beneficiaries from any racial or ethnic subgroup. Fourth, it would maximize the probability of cost savings or cost neutrality in Medicaid health care expenditures. Concerning the third objective—the absence of racial or ethnic bias—our conceptualization of group fairness was most closely aligned with what is commonly called demographic parity (4), which for this project was defined as racial or ethnic parity between eligible Medicaid beneficiaries experiencing homelessness and those selected by the algorithm.
Preliminary Algorithms
At the beginning of 2020, the ASO began receiving data on Medicaid beneficiaries who had stayed at least one night in a homeless shelter in the prior 3 months, as documented in the statewide HMIS. Merging HMIS and administrative Medicaid data yielded a cohort of 3,465 unique members who spent at least one night in a homeless shelter. The first set of algorithms drew on prior research on “impactability”—the likelihood that an intervention would reduce a particular person’s health care costs (8)—with a focus on identifying Medicaid beneficiaries with at least one general medical or psychiatric hospitalization and one potentially preventable rehospitalization. Additional efforts included developing models that compared the health care use of Medicaid beneficiaries experiencing housing insecurity with the health care use of Medicaid beneficiaries with stable housing, stratified by race and ethnicity. However, all these approaches to identifying potentially eligible individuals yielded cohorts that were too small for the annual target enrollment (the second objective) and that, more importantly, failed to meet the racial and ethnic fairness standard (the third objective). In Figure 1, the bars of algorithms 1 and 2 display the racial and ethnic disparities observed in these initial algorithms.
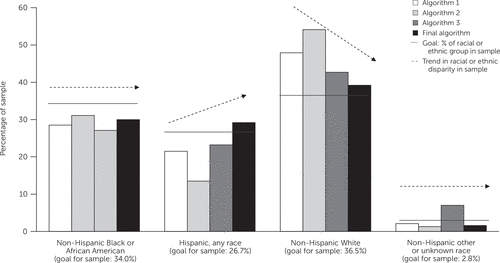
FIGURE 1. Racial and ethnic composition of samples across multiple iterations of an algorithm to select Medicaid enrollees experiencing homelessness or housing insecurity for an assistance program
Subsequent Algorithm
As we searched for alternative solutions while recognizing that a high percentage of individuals experiencing housing insecurity live with comorbid mental health conditions, substance use, and general medical disorders (9), the approach to assessing health risk shifted from health care use and expenditures to illness severity, with a focus on both general medical and behavioral health comorbid conditions. What resulted from this shift was a deprioritization of the fourth objective (maximizing the probability of cost savings or cost neutrality in Medicaid health care costs) and a greater emphasis on assessing health-related needs. After reviewing the literature and conferring with other researchers conducting similar work, we identified as an assessment tool the Combined Comorbidity Index (CCI), a validated index that combines two of the most popular comorbidity indices for use with administrative data: the Elixhauser Comorbidity Index (10) and the Charlson Comorbidity Index (11). The CCI identifies and categorizes different illnesses into diagnostic groups (e.g., hypertension, chronic obstructive pulmonary disease, diabetes, congestive heart failure, obesity, cerebrovascular disease, dementia, or severe mental illness) on the basis of prior health diagnoses.
We performed exploratory analyses, using the CCI to examine active illness severity in the prior 12 months, and identified two elements as potentially meaningful predictors for use in the revised algorithm: any behavioral health diagnosis and general illness severity as measured by the total CCI score (range –2 to 22, with higher scores representing greater acuity and health-related needs). For example, among Medicaid beneficiaries experiencing homelessness in the prior year, as identified in HMIS data, 79% (N=2,278 of 2,873) received a behavioral health diagnosis and 26.1% (N=751) had a CCI score of ≥3. These results would yield a potential outreach population of 729 Medicaid beneficiaries per year, far surpassing the goal of 150–170 per year. To reduce the size of the likely eligible population and to ensure that only those with a high level of clinical need were eligible, we increased the minimum CCI score from ≥3 to ≥4.
Although focusing the algorithm on comorbid conditions versus health care utilization increased the potential cost savings (the fourth objective) and reduced the racial and ethnic disparities to some extent (the third objective), the sample distribution of beneficiaries selected through the algorithm remained unfair and statistically significantly different from the subpopulation of Medicaid beneficiaries experiencing homelessness (χ2=113.88, df=3, p<0.001). Specifically, Blacks and Hispanics were underrepresented and non-Hispanic Whites were overrepresented (see the bars for algorithm 3 in Figure 1).
Final Algorithm
Further analyses and discussions with state partners and diverse stakeholders spurred us to resolve to think outside the box and incorporate non–health care data in order to improve the fairness of the algorithm. Adding the criterion of a Medicaid beneficiary spending at least 250 nights in a shelter in their lifetime helped to identify those most in need of housing and to mitigate the disproportionate overselection of non-Hispanic Whites and underselection of Hispanics. As can be seen in Figure 1, the final algorithm, which used as criteria the presence of a behavioral health diagnosis, a CCI score of ≥4, and ≥250 lifetime nights spent in a shelter, achieved fairness between racial and ethnic groups on the likelihood of an eligible individual being selected for the program. This selection process yielded no statistically significant differences in racial or ethnic representation between individuals who identified as non-Hispanic Black or African American, Hispanic of any race, non-Hispanic White, or non-Hispanic other or unknown race, compared with the total HMIS population. Moreover, this final algorithm maintained the sufficient outreach and potential cost savings achieved by the previous iteration.
Summary of the Algorithm Development Process
An overview of the iterative process that culminated in the development of an algorithm with no measurable racial or ethnic bias in the selection of Medicaid beneficiaries for program outreach is available in the online supplement to this column. Current machine-learning frameworks must underscore the importance of developing models that, for example, do not operate with racial-ethnic bias, and these models must not have a detrimental effect on outcomes for racial-ethnic minority subgroups (12). Stated differently, algorithmic fairness must guard protected groups so that similar individuals are treated similarly. Some of the primary ways we endeavored to achieve these aims were to change the algorithm’s inputs and outputs. With respect to inputs, we replaced health care service use with a measure of the severity of active comorbid general medical and behavioral health conditions, which are less susceptible to racial disparities (13). Moreover, adding an unconventional element (i.e., 250 or more lifetime shelter nights) to address a social determinant of health reduced many of the racial disparities that were present in prior iterations of the algorithm.
A crucial component of the process was engaging diverse stakeholders in a careful review of algorithm results. As part of a project advisory committee, persons with lived experience of homelessness, state Medicaid and government legal representatives, housing service providers, and advocacy organizations provided input and feedback on the project. Key issues were ensuring racial and ethnic parity of selected beneficiaries, adhering to Centers for Medicare and Medicaid Services policy and legal precedent regarding discrimination, and finding ways to expand the program while maintaining feasibility and cost neutrality. Stakeholders also helped to inform algorithm thresholds for program participation and practices for prioritizing self-applicants and allowing exceptions for individuals whose claims histories did not reflect their current health status. Work with the advisory committee led to deprioritizing cost savings and moving toward assessing health-related needs.
In addition to working closely with the advisory committee to reevaluate the evolving algorithm, our project team stayed abreast of current research. For example, our decision to change the inputs from health care use to severity of comorbid conditions was supported by the important and widely publicized work of Obermeyer et al. (2), which found that an extensively used algorithm believed to predict clinical risk (based on past health care costs rather than on health care needs) perpetuated and potentially exacerbated unfairness in health care.
Conclusions
Housing is a critical social determinant of health, and homelessness in the United States has been a long-standing and vexing challenge. Compared with people who have stable housing, people experiencing homelessness or housing insecurity face the intersection of physical, behavioral, and social burdens that are associated with significantly greater morbidity and mortality (9). Although the drivers of homelessness are complex and multifaceted, persons identifying as Black or African American are disproportionately affected and make up nearly 40% of the U.S. homeless population (14). Therefore, we felt that it was imperative that we develop an algorithm to select eligible Medicaid beneficiaries for coordinated housing and health care supports in a way that was fair and achieved racial and ethnic parity.
We came to three critical conclusions during the approximately 2-year effort to develop and refine the algorithm for this program. First, traditional measures of severity (e.g., health care cost and utilization) often lead to unfairness because they fail to characterize the severity of illness among persons of color as accurately as they do for White people. This conclusion is supported by a recent review of algorithms used in health care decisions (1). Second, switching to a diagnosis-based estimate of severity (e.g., a comorbidity index) can reduce but not eliminate algorithmic unfairness that is based on race or ethnicity. Again, this outcome has been observed in similar research (2). Finally, adding data on social determinants of health (i.e., days in a homeless shelter) as an algorithm input proved crucial in increasing the fairness of our algorithm’s output. By merging beneficiary-level administrative data from the HMIS with administrative health care data, we were able to effect racial and ethnic parity in our algorithm’s outputs, which will help redress unfairness in the deployment of homelessness assistance.
1. : Medical Algorithms Are Failing Communities of Color. Washington, DC, Health Affairs Forefront, 2021. https://www.healthaffairs.org/content/forefront/medical-algorithms-failing-communities-color. Accessed Jan 13, 2023 Google Scholar
2. : Dissecting racial bias in an algorithm used to manage the health of populations. Science 2019; 366:447–453Crossref, Medline, Google Scholar
3. Artificial Intelligence and Machine Learning in Software as a Medical Device. Silver Spring, MD, US Food and Drug Administration, 2024. https://www.fda.gov/medical-devices/software-medical-device-samd/artificial-intelligence-and-machine-learning-software-medical-device. Accessed May 31, 2024 Google Scholar
4. : A clarification of the nuances in the fairness metrics landscape. Sci Rep 2022; 12:4209Crossref, Medline, Google Scholar
5. : Evaluation and mitigation of racial bias in clinical machine learning models: scoping review. JMIR Med Inform 2022; 10:e36388Crossref, Medline, Google Scholar
6. : Housing as a determinant of health equity: a conceptual model. Soc Sci Med 2019; 243:112571Crossref, Medline, Google Scholar
7. All In: The Federal Strategic Plan to Prevent and End Homelessness. Washington, DC, US Interagency Council on Homelessness, 2022 Google Scholar
8. : Active redesign of a Medicaid care management strategy for greater return on investment: predicting impactability. Popul Health Manag 2018; 21:102–109Crossref, Medline, Google Scholar
9. : The health of homeless people in high-income countries: descriptive epidemiology, health consequences, and clinical and policy recommendations. Lancet 2014; 384:1529–1540Crossref, Medline, Google Scholar
10. : Comorbidity measures for use with administrative data. Med Care 1998; 36:8–27Crossref, Medline, Google Scholar
11. : New ICD-10 version of the Charlson Comorbidity Index predicted in-hospital mortality. J Clin Epidemiol 2004; 57:1288–1294Crossref, Medline, Google Scholar
12. : Ethical machine learning in healthcare. Annu Rev Biomed Data Sci 2021; 4:123–144Crossref, Medline, Google Scholar
13. : The intersection of homelessness, racism, and mental illness; in Racism and Psychiatry: Contemporary Issues and Interventions. Edited by Medlock MM, Shtasel D, Trinh NHT, . Cham, Switzerland, Humana Press, 2019 Crossref, Google Scholar
14. : The 2020 Annual Homeless Assessment Report (AHAR) to Congress. Washington, DC, US Department of Housing and Urban Development, 2021. https://www.huduser.gov/portal/sites/default/files/pdf/2020-ahar-part-1.pdf Google Scholar