A Latent Class Analysis of Age Differences in Choosing Service Providers to Treat Mental and Substance Use Disorders
Abstract
Objective
This study investigated choice of service provider for treatment of a mental or substance use disorder and its association with consumers’ age.
Methods
Data were from the Collaborative Psychiatric Epidemiology Surveys. Service users born between 1946 and 1964 were compared with those born in 1945 or earlier (N=4,082). Latent class analysis was used to identify groups of service users according to nine dichotomous items reflecting lifetime visits with different types of professionals. Multinomial logistic regression was used to analyze factors predicting latent class membership, with particular focus on both the direct and moderating effects of age. Analyses controlled for sociodemographic characteristics and disorder-related variables.
Results
Five classes of service user were identified. Class 1 (10.8%) included individuals who visited six of the nine types of providers (multiple providers visited). Class 2 (21.9%) had low probability of visiting most providers (limited providers visited). Class 3 (24.1%) visited a psychiatrist (primarily psychiatrist). Class 4 (28.1%) visited a family physician or other physician (primarily family physician). Class 5 (15.1%) visited a psychologist (primarily psychologist). A higher proportion of service users born before 1946 were in the primarily family physician class. Although 21% of service users born later also fell into this class, overall they were more evenly distributed across the five classes.
Conclusions
Family physicians played a significant role in behavioral health treatment for both age groups. However, findings suggest that younger adults may rely on more complex combinations of service providers that will require greater coordination between the behavioral and general health care systems in the future.
Older adults are more likely to visit a primary care physician for a behavioral health problem, and younger adults are more likely to seek help from a behavioral health specialist (1–5). Although service use may change with age, cohort differences have been found in both the prevalence of disorders and service use (6–8). These cohort differences are believed to persist even as changes occur with aging. Combined with the expected growth in the number of older adults overall, this finding suggests an increased need for physical and behavioral health services appropriate for older adults (9–13).
However, beyond documenting the greater use of primary care physicians, few studies have examined the combinations of service providers visited for behavioral health issues and how those combinations may vary by service users’ age. By developing typologies of service providers visited and grouping individuals reporting similar patterns, latent class analysis is a useful tool for examining the complexity and multidimensionality of service use (14). Studies that have taken this approach have contributed to a more nuanced understanding of service use that moves beyond classifications of users versus nonusers and focuses on identifying and describing subgroups of individuals. Such approaches can inform efforts to tailor interventions, outreach, and policy and facilitate the timely and effective use of behavioral health care.
For example, Choi and colleagues (15) identified three clusters of service use among older adults with severe depression. These clusters shed light on subgroups of this sample who used combinations of home-based supportive services for functional needs and outpatient behavioral health services to maintain their life in the community. Carragher and colleagues (16) examined three classes of service use for major depression (highly active, partially active, and inactive). Adults 65 and older were more likely to fall in the partially active class, members of which consulted a professional and received prescriptions for medication but had not been hospitalized or visited an emergency department for depression.
This study adds to this body of work in two ways. First, latent class analysis was used with nationally representative survey data to examine patterns of service providers visited for a behavioral health problem. Second, it examined how those patterns differ by age of service user.
Methods
Sample
This study used data from the Collaborative Psychiatric Epidemiology Surveys (CPES). Data were collected from 2001 to 2003 from three nationally representative surveys—the National Comorbidity Survey Replication (NCS-R), the National Survey of American Life (NSAL), and the National Latino and Asian American Survey (NLAAS). The NCS-R is representative of the U.S. population in general and included face-to-face interviews with 9,292 residents of English-speaking households who were 18 years or older. The NSAL is representative of blacks in the United States, and the survey was based on a national probability sample of 6,082 African Americans, blacks of Caribbean descent, and non-Hispanic whites. The NLAAS is a nationally representative sample of Latino and Asian populations in the United States, and the survey included 2,554 Latinos and 2,095 Asian Americans. The CPES surveys share a common set of objectives and instrumentation and are designed so that they can be combined as though they are a single, nationally representative study (17).
The analytic sample for this study included adults born in 1964 or earlier who reported using some professional services for a behavioral health problem (N=4,082). Those born between 1946 and 1964 and born earlier than 1946 encompass the baby boomer and preboomer generations, respectively, which were the focus of this study. Baby boomers made up 66% of the sample and 59.8% were female. Eighty-five percent of the sample was white, 7.9% African American, 5.7% Latino, 1.1% Asian, and .4% black Caribbean. After complete description of the study to participants, informed consent was obtained. All three CPES studies were approved by the University of Michigan Institutional Review Board.
Measures
Respondents were given a list of professionals and asked to indicate which ones they had seen in their lifetime “for problems with your emotions, nerves, or your use of alcohol or drugs.” Dichotomous indicators were created for visits with nine types of professional: psychiatrist; general practitioner, family physician, or other medical doctor; psychologist; social worker; counselor; any other mental health professional; a nurse, occupational therapist, or other health professional; a religious or spiritual advisor; or any other healer.
Other measures included age (born in 1945 or earlier or born between 1946 and 1964), gender, marital status (currently married, previously married, or never married), race-ethnicity (non-Hispanic white, African American, black Caribbean, Asian, or Latino), household income in quartiles ($0 to $16,699, $17,000 to $39,999, $40,000 to $73,498, or $73,499 and higher), years of education (≤11 years, 12 years, 13–15 years, or ≥16 years), work status (employed, unemployed, or not in labor force), insurance coverage (private, public, or none) and self-report of the presence of heart disease, arthritis, or diabetes. Mental and substance use disorders were assessed with the World Mental Health Composite International Diagnostic Interview, a structured, lay-administered diagnostic interview based on the definitions and criteria of ICD-10 and DSM-IV (18). On the basis of this assessment, dichotomous measures were created and indicated whether respondents met criteria for any lifetime mood disorder, anxiety disorder, or substance use disorder. Also included was a three-category measure of the number of disorders (none, one, and two or more).
Analyses
Latent class analysis was used to identify homogeneous groups of respondents on the basis of nine dichotomous items reflecting types of behavioral and health care professionals visited in their lifetime. Mplus (version 6) was used for the latent class analysis models, which included weights and complex survey design variables (19). In an effort to identify the smallest number of classes needed to account for patterns of service providers visited, the number of latent classes was determined iteratively, beginning with a one-class model and testing models of increasing numbers of classes with robust maximum likelihood estimation. The optimal number of classes was determined on the basis of several goodness-of-fit statistics (such as the Bayesian information criterion, Akaike information criterion, Lo-Mendell-Rubin’s adjusted likelihood ratio test, and entropy measures). The analysis also examined the extent to which classes were distinct and substantively meaningful (20).
Respondents were assigned to the class for which they had the highest probability of membership. For each class, the prevalence of respondents and the probability of visiting a particular service provider are reported. Conditional probabilities of .70–1.00 were considered to indicate a high probability of visiting a specific type of provider; .40–.69, a moderate probability; and <.40, a low probability (21,22).
After model estimation, multinomial logistic regression was used to analyze factors predicting latent class membership. These analyses were performed with the survey commands in Stata 12.0 (23), which accounted for the complex multistage clustered design of the CPES samples. All percentages reported are weighted. For each variable with more than two categories, a design-corrected Wald chi square test was conducted to minimize the likelihood of type I error resulting from multiple comparisons. An alpha of .05 was used as the cutoff for significance. Interactions between age and other predictors were also included to examine the moderating role of age.
Results
Compared with the younger adults, a higher proportion of the older adults was female and previously married (Table 1). Older adults were slightly less diverse by race-ethnicity and had fewer years of education. In contrast to the younger group, a majority of older adults were not in the labor force and had public health insurance coverage, and a higher proportion was in the lower two income quartiles. Fewer older adults met criteria for behavioral health disorders, whereas a higher proportion reported having general physical disorders. Compared with younger adults, a higher proportion of older adults visited a family or other physician for a behavioral health disorder. A higher proportion of the younger age group visited most other types of professionals.
Born prior to 1946(N=1, 328) | Born 1946 to 1964(N=2,754) | Total sample(N=4,082) | |||||||
---|---|---|---|---|---|---|---|---|---|
Characteristic | N | % | N | % | N | % | χ2b | df | p |
Gender | 8.75 | 1 | .004 | ||||||
Male | 435 | 35.8 | 1,019 | 42.4 | 1,454 | 40.2 | |||
Female | 893 | 64.2 | 1,735 | 57.6 | 2,628 | 59.8 | |||
Marital status | 35.78 | 2 | <.001 | ||||||
Currently married | 631 | 55.2 | 1,543 | 64.2 | 2,174 | 61.2 | |||
Previously married | 635 | 41.8 | 839 | 25.6 | 1,474 | 31.0 | |||
Never married | 62 | 3.0 | 372 | 10.2 | 434 | 7.8 | |||
Race-ethnicity | 6.96 | 4 | <.001 | ||||||
White | 806 | 87.9 | 1,391 | 83.6 | 2,197 | 85.0 | |||
African American | 271 | 6.6 | 691 | 8.6 | 962 | 7.9 | |||
Black Caribbean | 38 | .3 | 132 | .4 | 170 | .4 | |||
Asian | 47 | 1.2 | 103 | 1.0 | 150 | 1.1 | |||
Latino | 137 | 4.1 | 374 | 6.5 | 511 | 5.7 | |||
Education | 25.09 | 3 | <.001 | ||||||
≤11 years | 346 | 22.4 | 417 | 10.8 | 763 | 14.7 | |||
12 years | 388 | 33.0 | 732 | 29.3 | 1,120 | 30.6 | |||
13–15 years | 286 | 21.6 | 832 | 29.0 | 1,118 | 26.5 | |||
≥16 years | 308 | 23.0 | 773 | 30.8 | 1,081 | 28.2 | |||
Work status | 242.83 | 2 | <.001 | ||||||
Employed | 452 | 34.5 | 1,961 | 76.4 | 2,413 | 62.3 | |||
Unemployed | 125 | 11.2 | 164 | 4.0 | 289 | 6.5 | |||
Not in labor force | 746 | 54.3 | 617 | 19.6 | 1,363 | 31.3 | |||
Insurance coverage | 283.84 | 2 | <.001 | ||||||
Private | 370 | 38.2 | 1,629 | 83.6 | 1,999 | 68.9 | |||
Public | 628 | 61.3 | 487 | 15.1 | 1,115 | 30.1 | |||
None | 22 | .5 | 89 | 1.3 | 111 | 1.0 | |||
Household income | 12.09 | 3 | <.001 | ||||||
$0–$16,999 | 331 | 19.0 | 471 | 11.6 | 802 | 14.1 | |||
$17,000–$39,999 | 295 | 21.3 | 600 | 18.0 | 895 | 19.1 | |||
$40,000–$73,498 | 230 | 19.7 | 655 | 24.9 | 885 | 23.2 | |||
≥$73,499 | 448 | 39.9 | 987 | 45.6 | 1,435 | 43.7 | |||
Mood disorder | 354 | 27.5 | 1,008 | 39.5 | 1,362 | 35.4 | 25.58 | 1 | <.001 |
Anxiety disorder | 408 | 30.3 | 1,131 | 39.8 | 1,539 | 36.6 | 20.36 | 1 | <.001 |
Substance use disorder | 152 | 11.2 | 631 | 23.7 | 783 | 19.5 | 75.54 | 1 | <.001 |
Number of disorders | 36.14 | 2 | <.001 | ||||||
None | 653 | 51.0 | 988 | 36.1 | 1,641 | 41.1 | |||
1 | 371 | 26.1 | 683 | 25.1 | 1,054 | 25.4 | |||
≥2 | 304 | 22.9 | 1,083 | 38.8 | 1,387 | 33.5 | |||
Heart disease | 207 | 17.8 | 173 | 5.4 | 380 | 9.2 | 94.65 | 1 | <.001 |
Arthritis | 679 | 63.6 | 793 | 32.7 | 1,472 | 42.3 | 173.64 | 1 | <.001 |
Diabetes | 204 | 15.2 | 236 | 7.5 | 440 | 9.8 | 42.98 | 1 | <.001 |
Service provider visited | |||||||||
Psychiatrist | 450 | 32.1 | 989 | 33.8 | 1,439 | 33.2 | .83 | 1 | .364 |
Social worker | 82 | 5.4 | 356 | 12.8 | 438 | 10.3 | 39.96 | 1 | <.001 |
Counselor | 196 | 15.8 | 774 | 31.4 | 970 | 26.1 | 97.89 | 1 | <.001 |
Nurse or other health | 24 | 1.8 | 148 | 5.1 | 172 | 4.0 | 13.78 | 1 | <.001 |
Other mental health | 54 | 4.3 | 177 | 6.4 | 231 | 5.7 | 2.81 | 1 | .096 |
Religious or spiritual advisor | 186 | 13.5 | 542 | 19.8 | 728 | 17.7 | 22.08 | 1 | <.001 |
Healer | 22 | 1.5 | 112 | 4.2 | 134 | 3.3 | 19.78 | 1 | <.001 |
Psychologist | 282 | 21.4 | 817 | 32.5 | 1,099 | 28.8 | 23.40 | 1 | <.001 |
Family or other physician | 802 | 62.6 | 1,244 | 46.7 | 2,046 | 52.1 | 50.20 | 1 | <.001 |
Results of fitting latent class models
A five-class solution was chosen according to measures of model fit and because it was most conceptually meaningful. Figure 1 depicts the prevalence of each class and the predicted probability that service users assigned to a class would visit those specific service providers. Class 1, labeled “multiple providers visited,” contained 10.8% (N=419) of the respondents. Members of class 1 had a high probability of visiting a psychiatrist (.789) and a moderate probability of visiting a social worker (.427), a counselor (.611), a religious or spiritual advisor (.554), a psychologist (.670), or a family physician or other physician (.696). Members of this class had a low probability of visiting a nurse or other health professional (.219), another mental health professional (.233), or a healer (.180). Overall, members of class 1 had a moderate to high probability of visiting six out of nine types of providers.
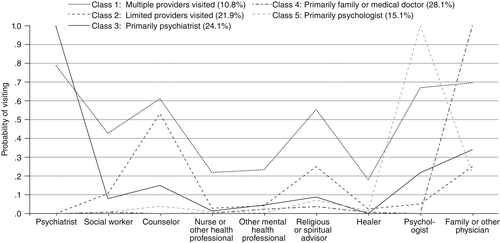
Class 2, labeled “limited providers visited,” contained 21.9% (N=878) of the respondents. Members of this group did not have a high probability of visiting any of the providers and had a moderate probability of visiting a counselor (.533). There was a low probability of visiting all other providers (≤.25) and no probability of visiting a psychiatrist. The defining characteristic of this group was low probability of visiting most providers.
Class 3, labeled “primarily psychiatrist,” contained 24.1% (N=1,093) of respondents. Members of this class had 100% probability of visiting a psychiatrist but low probability (≤.34) of visiting all other types of providers.
Class 4, labeled “primarily family physician,” contained 28.1% (N=1,128) of respondents. Members of this class had 100% probability of visiting a family physician or other physician and very low probability (≤.037) of visiting other professionals, including no probability of visiting a psychiatrist, counselor, or psychologist.
Finally, class 5, labeled “primarily psychologist,” contained 15.1% (N=564) of respondents. Members of this class had 100% probability of visiting a psychologist and low probability (≤.214) of visiting other types of providers, including no probability of visiting a psychiatrist.
Demographic and psychiatric predictors of class membership
The largest proportion of older adults (41.9%) was in the primarily family physician class, followed by primarily psychiatrist (25.8%), limited providers visited (13.5%), primarily psychologist (11.8%), and multiple providers visited (7.0%). Those in the younger age group were more evenly distributed, with roughly a quarter in the limited providers visited (26.1%), primarily psychiatrist (23.2%), and primarily family physician (21.2%) classes. Seventeen percent fell into the primarily psychologist class, and 12.8% fell into the multiple providers visited class (Rao-Scott χ2=38.07, df=4, p<.001).
Multinomial logistic regression was used to assess sociodemographic and disorder-related predictors of class membership, with the primarily family physician class as the reference category (Table 2). Older adults and women were less likely than the younger age group and men to be in every class compared with the primarily family physician class. Those who were previously married were more likely than those who were currently married to be in the limited providers class, whereas those who were previously or never married were more likely to primarily visit psychiatrists. More years of education was associated with a greater likelihood of being in every class compared with the primarily family physician class. Those who were unemployed were significantly more likely to be in the class that primarily visited psychiatrists, whereas those not in the labor force were more likely to visit multiple providers. Respondents with an anxiety disorder were less likely to be in the class that visited a limited number of providers, whereas those with a substance use disorder were more likely to have visited multiple providers or primarily psychiatrists. Compared with respondents who did not meet criteria for a behavioral health disorder, those with one disorder were more likely to be in the class with limited providers visited, whereas those with two or more disorders were more likely to have visited multiple providers or a limited number of providers.
Multiple providers visited | Limited providers visited | Primarily psychiatrist | Primarily psychologist | |||||
---|---|---|---|---|---|---|---|---|
Variable | RRR | 95% CI | RRR | 95% CI | RRR | 95% CI | RRR | 95% CI |
Born before 1946b | .35*** | .23–.52 | .38*** | .28–.51 | .62** | .45–.86 | .50** | .33–.75 |
Femaleb | .71* | .52–.97 | .72* | .53–.98 | .62** | .48–.82 | .61** | .40–.92 |
Marital status (reference: currently married)b | ||||||||
Previously married | 1.45 | .97–2.18 | 1.62** | 1.15–2.28 | 1.66*** | 1.25–2.20 | 1.10 | .77–1.57 |
Never married | 1.21 | .71–2.04 | .94 | .58–1.52 | 1.66* | 1.04–2.65 | .76 | .37–1.57 |
Race-ethnicity (reference: white)c | ||||||||
Asian | 1.97 | .62–6.27 | 1.16 | .49–2.75 | 2.00 | .78–5.13 | 1.64 | .44–6.10 |
Latino | .72 | .32–1.60 | 1.09 | .61–1.93 | .96 | .62–1.50 | .88 | .45–1.74 |
Black Caribbean | .23 | .05–1.03 | .86 | .23–3.18 | .42 | .15–1.18 | .67 | .21–2.14 |
African American | .85 | .57–1.29 | 1.38* | 1.00–1.91 | 1.20 | .91–1.59 | .65* | .44–.97 |
Education (reference: ≤11 years)b | ||||||||
12 years | 1.44 | .74–2.80 | 1.22 | .75–1.99 | 1.02 | .74–1.40 | 1.48 | .76–2.86 |
13–15 years | 3.03** | 1.63–5.64 | 1.63* | 1.01–2.64 | 1.49 | .99–2.26 | 2.46** | 1.26–4.81 |
≥16 years | 6.55*** | 3.31–12.94 | 2.96*** | 1.75–5.00 | 2.01** | 1.33–3.02 | 5.31*** | 2.66–10.59 |
Work status (reference: employed)b | ||||||||
Unemployed | 1.53 | .59–3.99 | .61 | .34–1.10 | 1.73* | 1.10–2.72 | 1.34 | .58–3.10 |
Not in labor force | 2.08* | 1.48–2.93 | 1.14 | .79–1.64 | 1.41 | .97–2.05 | 1.18 | .72–1.95 |
Insurance coverage (reference: private)c | ||||||||
Public | 1.16 | .68–1.96 | .77 | .48–1.22 | .91 | .69–1.19 | .73 | .38–1.40 |
None | .35 | .10–1.24 | 1.04 | .46–2.33 | .93 | .49–1.76 | .81 | .23–2.83 |
Household income (reference: 0 to $16,999)b | ||||||||
$17,000 to $39,999 | 1.14 | .68–1.90 | .79 | .53–1.19 | .89 | .61–1.30 | 1.29 | .57–2.91 |
$40,000 to $73,498 | 1.00 | .55–1.84 | 1.54 | .91–2.61 | 1.00 | .67–1.51 | 2.01 | .96–4.22 |
$73,499 and higher | .87 | .48–1.60 | 1.19 | .76–1.84 | 1.24 | .83–1.85 | 1.56 | .69–3.52 |
Mood disorderc | 1.63 | .96–2.80 | .93 | .59–1.46 | 1.38 | .78–2.46 | 1.21 | .79–1.85 |
Anxiety disorderb | 1.01 | .59–1.72 | .52* | .31–.89 | .85 | .52–1.39 | 1.05 | .59–1.85 |
Substance use disorderb | 1.77** | 1.26–2.48 | 1.42 | .98–2.05 | 1.60* | 1.07–2.40 | 1.40 | .83–2.36 |
Number of disorders (reference: none)b | ||||||||
1 | 1.71 | .82–3.55 | 1.71* | 1.05–2.80 | 1.24 | .74–2.07 | 1.00 | .55–1.82 |
≥2 | 4.00** | 1.76–9.12 | 2.46* | 1.18–5.15 | 1.61 | .74–3.50 | .76 | .31–1.86 |
Heart diseasec | .80 | .43–1.49 | .65 | .37–1.14 | 1.01 | .68–1.50 | .76 | .43–1.35 |
Arthritisc | 1.01 | .66–1.54 | .90 | .61–1.34 | 1.03 | .78–1.35 | .76 | .53–1.09 |
Diabetesc | .72 | .41–1.26 | .86 | .48–1.53 | 1.12 | .66–1.92 | 1.00 | .56–1.79 |
Analyses of interactions of age with other demographic variables indicated that older black Caribbeans were more likely than persons of other racial or ethnic groups to have visited a limited number of providers and to have visited primarily a psychiatrist (Table 3). Older adults who were not in the labor force, had public insurance coverage, or had no insurance coverage were less likely than younger adults to have visited multiple providers. Older adults with public insurance were also less likely to be in the classes with limited providers visited or visits primarily to psychiatrists. Older adults in the middle household income categories were more likely to be in the class that primarily visited psychologists, whereas those with incomes between $40,000 and $73,498 were also more likely to visit multiple providers.
Multiple providers visited | Limited providers visited | Primarily psychiatrist | Primarily psychologist | |||||
---|---|---|---|---|---|---|---|---|
Characteristic | RRR | 95% CI | RRR | 95% CI | RRR | 95% CI | RRR | 95% CI |
Black Caribbean | .62 | .06–6.64 | 7.91** | 1.74–36.04 | 3.45* | 1.05–11.38 | .52 | .07–3.98 |
Not in labor force | .24** | .10–.59 | 1.02 | .60–1.72 | .70 | .39–1.26 | .85 | .37–1.93 |
Insurance coverage | ||||||||
Public insurance | .26*** | .14–.50 | .53* | .28–.98 | .42*** | .26–.67 | .33 | .09–1.15 |
No insurance | .04* | .00–.54 | .25 | .04–1.48 | .28 | .07–1.09 | .57 | .04–7.40 |
Household income | ||||||||
$17,000–$39,999 | 2.03 | .98–4.19 | 2.01 | .71–5.71 | 1.50 | .58–3.87 | 4.45* | 1.04–19.0 |
$40,000–$73,498 | 3.71** | 1.64–8.41 | 2.28 | .59–8.85 | 1.64 | .80–3.34 | 4.51* | 1.14–17.75 |
Discussion
Consistent with previous research, this study indicated that when faced with a behavioral health problem, older adults relied heavily on a family physician or other physician within the general medical sector (1–5). Furthermore, older adults were more likely than the younger age group to rely primarily on a family physician compared with every other class of provider, suggesting that baby boomers visited a broader range of providers. Although it is not possible to disentangle aging from cohort effects with these cross-sectional data, previous research with longitudinal data has found age cohort differences in service use that have remained significant over time, suggesting the possibility that differences found in this study may continue with age (6). It is reasonable to expect that the use of the general medical sector will increase as baby boomers encounter more general health problems with age. However, cohort-specific cultural and social differences, such as a greater acceptability of reporting and seeking treatment for behavioral health problems, may continue to influence service use patterns (7,24).
Efforts have been made to integrate behavioral health treatment into primary care with the explicit objective of improving treatment for older adults (25,26). Findings from this study support the continued importance of these efforts. Almost half of the younger age group (46.7%) reported visiting a family physician or other physician for a behavioral health problem, and 21.0% were in the primarily family physician class. Primary care physicians clearly play an important role in behavioral health care. Additional research is needed to clarify whether visits to different providers occur simultaneously or concurrently and the gateways through which individuals enter services, as well as patterns of referral and the extent to which treatment is coordinated across service providers. However, this study reaffirmed the importance of ongoing efforts to better connect behavioral and general medical care.
Several other differences by age are worthy of note. Although insurance coverage did not have a main effect on class membership, older adults with public insurance coverage were less likely than the younger group to fall into three of the five classes. One possible reason for this is that Medicare, the public insurance by which most older adults are covered, does not cover services from many of the other providers considered in this study. Second, those in the younger group were more likely to be covered by Medicaid, which is need based, whereas Medicare is universal for those ages 65 and over. Unfortunately, the data did not allow differentiation of insurance coverage at this level, limiting the ability to separate age differences from differences in coverage or to examine some of the complexities of coverage, such as dual eligibility for both Medicare and Medicaid.
Although there were few racial-ethnic differences overall, older black Caribbeans were more likely to be in the class that visited a limited number of providers. Previous studies have found that persons from racial-ethnic minority groups are more likely than non-Hispanic whites to use the general medical sector rather than specialty care for a behavioral health problem (1–3,27,28). Overall, this study suggests that, among service users, when a range of service providers is considered, the relationship between race-ethnicity and where people go for help is more nuanced. It also highlights the importance of considering within- as well as between-group differences. Black Americans are often treated as a homogeneous group, although blacks of Carribean descent and African Americans differ in terms of ethnicity, national heritage, socioeconomics, and immigration status (29). In combination with other recent studies, these findings highlight the importance of looking further at within-group heterogeneity (2,30,31). Such complexities may influence treatment outcomes and pathways into and out of care.
The effect of employment status on class membership was also moderated by age. For the sample as a whole, not being in the labor force was associated with visiting multiple providers. However, older adults who were not in the labor force were significantly less likely to fall into this class. This may in part be due to individuals’ reasons for not being in the labor force. Among the younger age group, for example, those not in the labor force were more likely to have multiple disorders compared with those who were employed or unemployed, suggesting an association between severity of disorders and work status. Older adults, over half of whom were not in the labor force, may be in retirement.
One major limitation of this study is the cross-sectional nature of the data. For this reason, it was not possible to determine the extent to which respondents visited service providers simultaneously or serially nor how the combinations of service providers visited may have changed over time. Cross-sectional data also limit the ability to differentiate cohort versus aging effects as described above. Furthermore, these data were collected between 2001 and 2003. Over the past decade, significant changes have occurred in the financing and delivery of behavioral health services, including the increased use of managed care and behavioral health parity legislation (32,33). Previous studies suggest that parity in behavioral health care coverage can increase the use of appropriate services (33); however, research has not examined the influence of systemic changes on the types of providers visited. With time, we will learn whether perceived cohort differences suggested by this and other research remain in the face of such structural changes.
Conclusions
Despite these limitations, this study begins to shed more light on the combinations of service providers most often visited. The findings provide further evidence of the underutilization of behavioral health specialty services by older adults. Although younger adults, compared with older adults, relied on a broader array of professionals for treatment of behavioral health care problems, primary care physicians played a significant role in their care. As this younger cohort ages and requires additional medical treatment, the need to coordinate general medical and behavioral health services will grow. Increasing our understanding of the current complexities of service provider usage can inform ongoing and future efforts in this area.
1 : Twelve-month use of mental health services in the United States: results from the National Comorbidity Survey Replication. Archives of General Psychiatry 62:629–640, 2005Crossref, Medline, Google Scholar
2 : Race, ethnicity, and the use of services for mental disorders: results from the National Survey of American Life. Archives of General Psychiatry 64:485–494, 2007Crossref, Medline, Google Scholar
3 : Mental health service use among older African Americans: the National Survey of American Life. American Journal of Geriatric Psychiatry 16:948–956, 2008Crossref, Medline, Google Scholar
4 : Use of mental health care by community-dwelling older adults. Journal of the American Geriatrics Society 59:50–56, 2011Crossref, Medline, Google Scholar
5 : Serious psychological distress and mental health service use among community-dwelling older US adults. Psychiatric Services 62:291–298, 2011Link, Google Scholar
6 : Mental health services use: Baltimore Epidemiologic Catchment Area follow-up. American Journal of Geriatric Psychiatry 17:706–715, 2009Crossref, Medline, Google Scholar
7 : Baby boomers nearing retirement: the healthiest generation? Rejuvenation Research 13:105–114, 2010Crossref, Medline, Google Scholar
8 : Projecting drug use among aging baby boomers in 2020. Annals of Epidemiology 16:257–265, 2006Crossref, Medline, Google Scholar
9 : The 2030 problem: caring for aging baby boomers. Health Services Research 37:849–884, 2002Crossref, Medline, Google Scholar
10 : Annual incidence of Alzheimer disease in the United States projected to the years 2000 through 2050. Alzheimer Disease and Associated Disorders 15:169–173, 2001Crossref, Medline, Google Scholar
11 : Substance abuse treatment need among older adults in 2020: the impact of the aging baby-boom cohort. Drug and Alcohol Dependence 69:127–135, 2003Crossref, Medline, Google Scholar
12 : The prevalence of mental and physical health disorders among older methadone patients. American Journal of Geriatric Psychiatry 16:488–497, 2008Crossref, Medline, Google Scholar
13 : Social and cultural constructions of ageing: the case of the baby boomers. Sociological Research Online 13(3):5, 2008Crossref, Google Scholar
14 : Latent variable modeling in epidemiology. Alcohol Health and Research World 16:286–292, 1992Google Scholar
15 : Configuration of services used by depressed older adults. Aging and Mental Health 10:240–249, 2006Crossref, Medline, Google Scholar
16 : Treatment-seeking behaviours for depression in the general population: results from the National Epidemiologic Survey on Alcohol and Related Conditions. Journal of Affective Disorders 121:59–67, 2010Crossref, Medline, Google Scholar
17 Heeringa SG, Berglund P: Collaborative Psychiatric Epidemiology Survey Program (CPES) Data Set: Integrated Weights and Sampling Error Codes for Design-Based Analysis. Rockville, Md, National Institute of Mental Health, 2007. Available at www.icpsr.umich.edu/icpsrweb/CPES/about_cpes/weighting/index.jspGoogle Scholar
18 : The World Mental Health (WMH) Survey Initiative Version of the World Health Organization (WHO) Composite International Diagnostic Interview (CIDI). International Journal of Methods in Psychiatric Research 13:93–121, 2004Crossref, Medline, Google Scholar
19 : Mplus: Statistical Analysis With Latent Variables User’s Guide. Los Angeles, Muthen & Muthen, 2001Google Scholar
20 : Deciding on the number of classes in latent class analysis and growth mixture modeling: a Monte Carlo simulation study. Structural Equation Modeling 14:535–569, 2007Crossref, Google Scholar
21 : Patterns of perceived barriers to medical care in older adults: a latent class analysis. BMC Health Services Research 11:181, 2011Crossref, Medline, Google Scholar
22 : Latent Class and Latent Transition Analysis: With Applications in the Social, Behavioral, and Health Sciences. Hoboken, NJ, Wiley, 2010Google Scholar
23 Stata Statistical Software: Release 12. College Station, Tex, StataCorp, 2011Google Scholar
24 : Substance abuse among aging baby boomers: health and treatment implications. Journal of Addictions Nursing 20:124–126, 2009Crossref, Google Scholar
25 : Collaborative care management of late-life depression in the primary care setting: a randomized controlled trial. JAMA 288:2836–2845, 2002Crossref, Medline, Google Scholar
26 : Reducing suicidal ideation in depressed older primary care patients. Journal of the American Geriatrics Society 54:1550–1556, 2006Crossref, Medline, Google Scholar
27 : The epidemiology of major depressive disorder: results from the National Comorbidity Survey Replication (NCS-R). JAMA 289:3095–3105, 2003Crossref, Medline, Google Scholar
28 : Racial/ethnic discrimination and health: findings from community studies. American Journal of Public Health 93:200–208, 2003Crossref, Medline, Google Scholar
29 Logan JR, Deane G: Black Diversity in Metropolitan America. Albany, State University of New York, Mumford Center for Comparative Urban and Regional Research, 2003. Available at mumford1.dyndns.org/cen2000/BlackWhite/BlackDiversityReport/black-diversity01.htmGoogle Scholar
30 : Prevalence and distribution of major depressive disorder in African Americans, Caribbean blacks and non-Hispanic whites: results from the National Survey of American Life. Archives of General Psychiatry 64:305–315, 2007Crossref, Medline, Google Scholar
31 : Differences in professional and informal help-seeking among older African Americans, black Caribbeans, and non-Hispanic whites. Journal of the Society for Social Work and Research 1:124–139, 2010Crossref, Medline, Google Scholar
32 : Policy issues in mental health among the elderly. Nursing Clinics of North America 45:627–634, vii, 2010Crossref, Medline, Google Scholar
33 : Insurance parity and the use of outpatient mental health care following a psychiatric hospitalization. JAMA 300:2879–2885, 2008Crossref, Medline, Google Scholar