Assessing Risk of Future Violence Among Forensic Psychiatric Inpatients With the Classification of Violence Risk (COVR)
Understanding the potential for future disruptive and violent behavior of an individual is important for appropriate allocation of limited resources and formulation of a treatment and management plan. There is great pressure to ensure that any such judgment is evidence based, rather than based on pure clinical judgment, which is thought to be unreliable ( 1 , 2 ). Therefore, many attempts have been made to produce instruments that will aid the clinician in making judgments about future violence.
The Classification of Violence Risk (COVR) ( 3 ) was developed from the MacArthur Violence Risk Assessment Study in the United States ( 1 ). The MacArthur Study measured many variables in a sample of more than 1,000 acute psychiatric patients who had been civilly committed and followed them for 20 weeks after discharge to the community. Variables that were predictive of future violence were then used to classify study participants into risk categories via a classification tree analysis ( 4 ). This type of analysis allowed for nonlinear interactions between variables associated with violence, whereas many other models that are based on main effects do not (for example, the Violence Risk Appraisal Guide [VRAG] [ 5 ]). The classification tree showed outstanding prediction of violence in the construction sample (area under the curve [AUC]=.82) ( 6 ).
However, data for many of the variables used in the MacArthur Study analysis, such as the psychopathy score, are unlikely to be readily available in many clinical settings. To maximize ease of use of the COVR for busy clinicians, its developers omitted any risk indicators that were unlikely to be available in hospital records or that could not be easily gathered in a clinical interview. Using the remaining risk indicators, the investigators were still able to predict violence with a high level of accuracy (AUC=.80).
In devising a risk assessment instrument based on empirical data, some loss of power is likely when it is applied to a new (but similar) population. The reason for the "shrinkage" is that the original model takes advantage of chance associations between risk indicators and outcomes that may not exist in the new sample. Therefore, Monahan and colleagues ( 7 ) applied the COVR to a new sample of civilly committed psychiatric patients and followed a subsample of these individuals (102 low-risk patients and 55 high-risk patients). The results did show some slippage, with an initial AUC of .63, which was revised to an AUC of .70 when a slightly more inclusive measure of violence was used.
Thus the COVR appears to have potential as an easy-to-use instrument that can be quickly administered and that can aid clinicians in their judgment of violence risk. However, it has been applied only to the samples selected by the COVR authors—that is, to civil psychiatric patients discharged to the community in the United States. In this study we undertook the first independent test of the COVR. We also conducted a severe test of its predictive accuracy by using it in a sample that differed from the construction sample in three important ways: our sample included forensic psychiatric patients, who resided in secure inpatient psychiatric units and in the United Kingdom.
Methods
Design
The study was a prospective analysis of patients residing in medium-security forensic psychiatric units in the United Kingdom. Two risk assessment instruments (COVR and VRAG) were completed for each patient. Information about incidents that occurred in the unit over the next six months was obtained from the contemporaneous nursing records.
Participants
Patients resided in one of four medium-security units. Two are run by the National Health Service (NHS), and the other two are run by Partnerships in Care Ltd, an independent health care provider. Data were collected over the period of 2006–2008. This article reports on patients for whom we successfully gathered all required data sets (COVR, VRAG, and follow-up data). The sample consisted of 52 patients (44 men) with a mean age of 34.0±10.5 years. Most patients (N=48, 92%) were Caucasian. Primary diagnoses were schizophrenia or psychotic disorder (N=24, 46%), mental retardation (N=18, 35%), personality disorder (N=7, 13%), affective disorder (N=2, 4%), and "other" diagnoses (N=1, 2%; anxiety disorder, developmental disorder, organic disorder, and epilepsy). Diagnoses were made at hospital admission by a consultant psychiatrist using ICD-10 criteria ( 8 ).
Measures
The COVR guides the clinician through a series of questions related to the presence or absence of risk indicators for the patient ( 3 ). COVR software places a person into one of five possible risk categories ranging from very low to very high. The VRAG ( 5 ) is a well-established actuarial instrument for the prediction of violence. It has an impressive record of violence prediction among various groups, including forensic psychiatric patients in the United Kingdom ( 9 ), civilly committed psychiatric patients ( 10 ), and inpatients ( 11 ).
Any violent behavior recorded in the continuous care records in the six months after the COVR and VRAG were completed was logged with the Aggression Vulnerability Scale (AVS) ( 12 ). Intraclass correlations, obtained by comparison of the scores of the two raters on a subsample of ten patients, were .99 for verbal aggression, .79 for aggression against property, and .92 for physical aggression.
Procedure
The NHS Multi-Centre Research Ethics Committee (MREC) and the Ethical Committee of the School of Psychology, Cardiff University, approved the study. Written informed consent was obtained after the procedures had been fully explained to each participant. Two raters from the study team completed the COVR and VRAG for each patient after a review of all pertinent files (for example, medical and criminal records) and an interview with the patient. Medical staff were kept blind to the results of the COVR and the VRAG so that the risk assessments could not influence patient treatment and management.
Analysis
The relationships between the instrument scores and the behavioral outcome measures were analyzed by use of correlational techniques and by the receiver operating characteristic (ROC) ( 13 ) with SPSS, version 12.0.1. ROC plots the sensitivity of the test (proportion of correct predictions that the behavior will occur) against 1 minus the specificity of the test (proportion of incorrect predictions that the behavior will occur) for each possible score of the instrument. The resulting curve can be quantified via the AUC. Instruments that have no predictive quality produce AUCs of .5, whereas perfect predictors produce AUCs of 1.0.
Results
Risk scores
Figure 1 shows the proportions of our sample in each of the five COVR risk categories, along with the proportions in the construction sample of Monahan and colleagues ( 1 ). Our population differs from the construction sample, especially in the relatively small percentage of participants in our sample who were in the lowest risk category. This is not surprising because our sample comprised forensic patients and the construction sample comprised civilly committed psychiatric patients. Antisocial behavior, including violent behavior, was very prevalent in the histories of patients in our sample. In a similar vein, the mean±SD VRAG score in our sample was 9.7±9.8, indicating an overall high score (greater violence risk) and an absence of anyone with a very low score. (Possible scores range from -26 to 38, with higher scores indicating a higher level of violence risk.) The COVR and VRAG scores were significantly correlated (r=.58, p<.001).
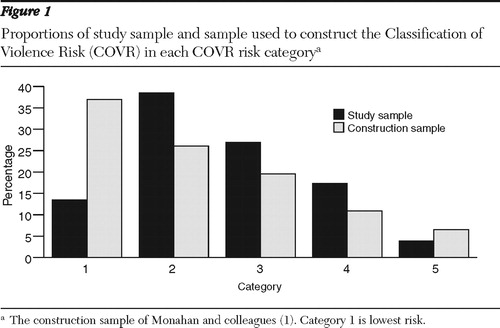
Aggressive behaviors
Every person in our sample committed at least one act of verbal aggression, with a mean of 10.0±15.0 incidents per 100 days (range .3–55.7). The mean rate for aggression toward property was 1.7±4.4 incidents per 100 days (range 0–14.8), and the rate for physical aggression was 1.2±3.5 (range 0–9.3).
Accuracy of risk prediction
Table 1 presents the bivariate correlations between rates of aggressive behaviors and scores on the risk assessment instruments, as well as the AUC of the ROC ( 13 ) for predicting whether an individual engaged in any incidents within the six-month period (this was not calculated for verbal aggression because every individual had at least one incident). It is clear that the COVR was a good predictor of both verbal and, most crucially, physical aggression. The VRAG was a good predictor of all forms of aggressive behaviors. There were no significant differences in the ability of the two instruments to predict verbal or physical aggression, but the VRAG was a better predictor of property aggression ( 14 ).
![]() |
Discussion
This is the first report of an independent validation of the COVR. The finding of good accuracy of prediction is all the more remarkable given that the COVR was developed with a sample of civilly committed psychiatric patients discharged to North American communities. Our study differs from that study in two major ways—our sample was of forensic psychiatric patients and their aggressive behaviors as inpatients were examined—and one minor way—it was conducted in the United Kingdom rather than the United States. Below we discuss the implications of these differences.
Forensic psychiatric setting
Patients in forensic psychiatric settings differ from those in civil settings in both the frequency and the severity of violent behaviors. Predicting future violence in forensic settings is difficult because patients are often concerned about factors that might influence their release or privileges and thus may not be honest in reporting their behaviors or intentions. Furthermore, one of the best predictors of future violence is past violent behaviors ( 15 ). However, in forensic settings this is not a very distinguishing risk indicator—at least when it is treated as simply present or absent—because most individuals have such a history. Despite these limitations, the COVR proved to be a good predictor in this setting, and thus this study provides the first evidence of its usefulness in forensic services.
Institutional setting
Predicting who will be violent in a secure setting is important given the limited resources available to manage large groups of inmates or patients. Institutional violence may not generate as much media attention as violence committed in the community. However, violence in institutional settings is still of great importance because of its effects on staff members, staff morale, and the safety of other inmates and its consequences for the perpetrator. The finding that the COVR can predict violence in institutional settings is important. Most such institutions now practice individual risk management. The COVR's ability to identify persons who are more dangerous will help institutions reduce the incidence of aggressive behavior and contribute to their risk management efforts. Therefore, the COVR's risk prediction ability demonstrated in this study can be seen as a lower limit of its efficacy.
United Kingdom and other European settings
The COVR was found to predict future violence in a U.K. setting. Thus the study provides evidence of the instrument's usefulness in clinical and forensic decision making in the United Kingdom. The results, however, are not particularly surprising because several other instruments developed in North America have demonstrated equal predictive validity in the United Kingdom ( 9 , 11 , 1617 ,18) and in other European settings ( 19 , 20 , 21 , 22 ). The implication is that risk factors for future violence do not differ greatly between populations in various countries, although studies are needed to validate the COVR's predictive validity with regard to gender and to racial-ethnic minority groups in North America and in Europe.
Comparison with other risk assessment instruments
In this study we also used the VRAG to predict violence. We chose this instrument because it has a good track record of prediction in many settings, including the United Kingdom ( 9 ), and in institutional settings ( 11 ). Our findings confirm the predictive abilities of the VRAG. It was a better predictor than the COVR of violence to property, but the two instruments were about equal in predicting verbal aggression and physical aggression toward others. The VRAG's slightly better performance might be expected because it was developed with a sample of forensic patients, whereas the COVR was developed with a sample of civilly committed patients.
Much larger samples are needed to determine whether one of these instruments is better when used in a given setting. A more pertinent question might address which of them to choose if we accept that both have good—and approximately equal—risk prediction ability. The COVR was designed to use data that are readily available in most settings and from a brief interview. It is scored automatically by the COVR software, which eliminates a source of possible error (although it would not be difficult to develop such a program to score the VRAG). On the other hand, the VRAG leans heavily on information that is not always easy to gather. For instance, the psychopathy score has the greatest predictive value of any of the VRAG items, but to obtain this score one must complete the Hare Psychopathy Checklist-Revised (PCL-R) ( 23 ) or a similar instrument. The PCL-R requires a lengthy interview and a file review and must be completed by a trained assessor. Indeed, it has been estimated that it may take days to complete a VRAG ( 24 ), whereas the COVR takes only ten minutes. We estimate that depending on the amount of collateral material, the COVR takes 15 minutes compared with three hours for the VRAG. Thus, for some uses, such as for screening large samples, the COVR may have distinct practical and financial advantages over the VRAG.
Limitations
The COVR requires a mixture of information from files and patient self-reports. The reliability of self-report is limited (for example, lack of insight into one's behavior), and the limitations are particularly acute among forensic patients, who have a vested interest in appearing "low risk" on assessment. In addition, forensic patients may be unreliable when it comes to self-reports of past violence. In some cases, information from a patient's self-report differs from that in the files. The COVR manual recommends confronting the patient with the discrepancy and coming to an agreement on the truth, or if this is not possible, marking the item as "missing" ( 3 ). However, we did not find this easy to do in our forensic population, and following this procedure would have resulted in loss of many assessments because of missing data. Instead, we asked the clinician to judge which information was correct. The COVR manual suggests that use of clinician judgment is "the more empirically appropriate strategy" for patients who may have good reason for poor self-disclosure. Our findings indicate that it is an effective method for completing the COVR in forensic settings.
The COVR provides a statement of risk couched in terms of descriptions of the violence risk category (for example, high risk), probability (for example, 56% chance), and frequency (for example, 56 people out of 100 in this category). However, the eventual aim of risk assessment is not to generate a label or a number for an individual but to manage and treat the person in a way that will minimize violence risk and other risks. Thus the COVR does not provide much information about why a person is high or low risk and what can be done about it. Indeed, the nature of the nonlinear interactions inherent in a tree structure would make it difficult to identify ways to address risk even if an attempt was made to do so. The authors of the COVR acknowledge this: they clearly state that the COVR is a tool to help inform clinical decision making and is not the decision-making process itself.
Finally, the findings of this study are for a small sample in a particular type of forensic institution (medium-security unit) in the United Kingdom. Although the results are encouraging in themselves, we hope they will also spur others to test this instrument in other settings and in other populations.
Conclusions
The results provide an evidence-base for the use of COVR in predicting violence in forensic inpatient settings.
Acknowledgments and disclosures
Partnerships in Care provided funding for the completion of this study.
The authors report no competing interests.
1. Monahan J, Steadman HJ, Silver E, et al: Rethinking Risk Assessment: The Macarthur Study of Mental Disorder and Violence. New York, Oxford University Press, 2001Google Scholar
2. Gardner W, Lidz CW, Mulvery EP, et al: Clinical versus actuarial predictions of violence in patients with mental illness. Journal of Consulting and Clinical Psychology 64:602–609, 1996Google Scholar
3. Monahan J, Steadman HJ, Appelbaum PS, et al: Classification of Violence Risk (COVR). Lutz, Fla, Psychological Assessment Resources, 2005Google Scholar
4. Breiman L, Friedman J, Olshen R, et al: Classification and Regression Trees. Boca Raton, Fla, CRC Press, 1984Google Scholar
5. Quinsey VL, Harris GT, Rice ME, et al: Violent Offenders: Appraising and Managing Risk, 2nd ed. Washington, DC, American Psychological Association, 2006Google Scholar
6. Monahan J, Steadman HJ, Appelbaum PS, et al: Developing a clinically useful actuarial tool for assessing violence risk. British Journal of Psychiatry 174:312–319, 2000Google Scholar
7. Monahan J, Steadman HJ, Robbins PC, et al: An actuarial model of violence risk assessment for persons with mental disorders. Psychiatric Services 56:810–815, 2005Google Scholar
8. The ICD-10 Classification of Mental and Behavioral Disorders. Geneva, World Health Organization, 1992Google Scholar
9. Snowden RJ, Gray NS, Taylor J, et al: Actuarial prediction of violent recidivism in mentally disordered offenders. Psychological Medicine 37:1539–1549, 2007Google Scholar
10. Harris GT, Rice ME, Camilleri JA: Applying a forensic actuarial assessment (the Violence Risk Appraisal Guide) to nonforensic patients. Journal of Interpersonal Violence 19:1063–1074, 2004Google Scholar
11. Doyle M, Dolan M, McGovern J: The validity of North American risk assessment tools in predicting in-patient violent behaviour in England. Legal and Criminological Psychology 7:141–154, 2002Google Scholar
12. Gray NS, Hill C, McGleish A, et al: Prediction of violence and self-harm in mentally disordered offenders: a prospective study of the efficacy of HCR-20, PCL-R and psychiatric symptomatology. Journal of Consulting and Clinical Psychology 71:443–451, 2003Google Scholar
13. Green DM, Swets JA: Signal Detection Theory and Psychophysics. New York, Wiley, 1966Google Scholar
14. Hanley JA, McNeil BJ: A method of comparing the areas under the receiving operating characteristic curves derived from the same cases. Radiology 148:839–843, 1983Google Scholar
15. Bonta J, Law M, Hanson K: The prediction of criminal and violent recidivism among mentally disordered offenders: a meta-analysis. Psychological Bulletin 123:123–142, 1998Google Scholar
16. Gray NS, Snowden RJ, MacCulloch S, et al: Relative efficacy of criminological, clinical and personality measures of future risk of offending in mentally disordered offenders: a comparative study of HCR-20, PCL:SV and OGRS. Journal of Consulting and Clinical Psychology 72:523–530, 2004Google Scholar
17. Gray NS, Taylor J, Snowden RJ. Predicting violent reconvictions using the HCR-20. British Journal of Psychiatry 192:384–387, 2008Google Scholar
18. Doyle M, Dolan M: Predicting community violence from patients discharged from mental health services. British Journal of Psychiatry 189:520–526, 2006Google Scholar
19. Belfrage H, Fransson G, Strand S: Prediction of violence using the HCR-20: a prospective study in two maximum security correctional institutions. Journal of Forensic Psychiatry 11:167–175, 2000Google Scholar
20. Pham TH, Ducro C, Marghem B, et al: Prediction of recidivism among prison inmates and forensic patients in Belgium. Annales Medico-Psychologiques 163:842–845, 2005Google Scholar
21. Grann M, Belfrage H, Tengstrom A: Actuarial assessment of risk for violence: predictive validity of the VRAG and the historical part of the HCR-20. Criminal Justice and Behaviour 27:97–114, 2000Google Scholar
22. Urabaniok F, Noll T, Grunewald S, et al: Prediction of violent and sexual offences: a replication study of the VRAG in Switzerland. Journal of Forensic Psychiatry and Psychology 17:23–31, 2006Google Scholar
23. Hare RD: The Hare Psychopathy Checklist-Revised (PCL-R), 2nd ed. Toronto, Multi-Health Systems, 2003Google Scholar
24. Stefan S. Emergency Department Treatment of the Psychiatric Patient: Policy Issues and Legal Requirements. Oxford, United Kingdom, Oxford University Press, 2007Google Scholar