Patient Feedback on the Use of Predictive Analytics for Suicide Prevention
Abstract
Objective:
There is significant debate about the feasibility of using predictive models for suicide prevention. Although statistical considerations have received careful attention, patient perspectives have not been examined. This study collected feedback from high-risk veterans about the U.S. Department of Veterans Affairs (VA) prevention program called Recovery Engagement and Coordination for Health–Veterans Enhanced Treatment (REACH VET).
Methods:
Anonymous questionnaires were obtained from veterans during their stay at a psychiatric inpatient unit (N=102). The questionnaire included three vignettes (the standard VA script, a more statistical vignette, and a more collaborative vignette) that described a conversation a clinician might initiate to introduce REACH VET. Patients rated each vignette on several factors, selected their favorite vignette, and provided qualitative feedback, including recommendations for clinicians.
Results:
All three vignettes were rated as neutral to very caring by more than 80% of respondents (at least 69% of respondents rated all vignettes as somewhat caring to very caring). Similar positive feedback was obtained for several ratings (e.g., helpful vs. unhelpful, informative vs. uninformative, encouraging vs. discouraging). There were few differences in the ratings of the three vignettes, and each of the three scripts was preferred as the “favorite” by at least 28% of the sample. Few patients endorsed concerns that the discussion would increase their hopelessness, and privacy concerns were rare. Most of the advice for clinicians emphasized the importance of a patient-centered approach.
Conclusions:
The results provide preliminary support for the acceptability of predictive models to identify patients at risk for suicide, but more stakeholder research is needed.
HIGHLIGHTS
Veterans at high risk for suicide reported that a variety of approaches to introducing a predictive-model suicide prevention program were caring, helpful, encouraging, and informative.
Negative patient reactions (e.g., concerns about a privacy violation, increased hopelessness) were rare.
Veterans’ advice to clinicians emphasized the importance of using a patient-centered approach that is respectful and caring during discussions with patients.
The growth of massive data storage solutions, computing power, and advanced statistical techniques (e.g., machine learning) is transforming many areas of life, including entertainment, advertising, and commerce (1). The health care sector is working to take advantage of this progress as well, especially with the advent of the electronic health record (2). The number of medical publications with a focus on machine learning increased from about 1,700 to almost 4,000 from 2016 to 2018 (3).
Predictive analytics have also generated excitement in the field of suicide prevention. Recent research has suggested that new paradigms and novel approaches to suicide prevention are desperately needed. The suicide rate has increased 33% in the United States since 1999 (4), and there are intense public concerns about high suicide rates among some special populations, such as military personnel and veterans (5). In addition, the use of traditional suicide risk factors and warning signs to predict risk is not especially helpful (6). Therefore, the promise of predictive models for suicide prevention has generated significant interest (7).
There is a serious debate, however, about the feasibility of predictive models for suicide prevention. Some researchers have argued forcefully that the low base rate of suicide will inevitably result in very low positive predictive values for any predictive model (8). Others have argued that very helpful interventions are still possible for models with a low positive predictive value because the net benefit of an intervention can still be positive (9). Still others have noted that although prediction of suicide may be inherently challenging, the feasibility for other suicide behaviors (e.g., suicide attempts) is high because the base rates of such behaviors are much higher (i.e., higher positive predictive values are possible) (10). Therefore, research on what may be helpful is progressing.
Absent from the discussion in the literature thus far is the patient perspective. Ethical analyses have raised concerns about how patients might view suicide prediction. Tucker et al. (11) noted that “research is needed to guide providers in how (and whether) to discuss suicide prediction results with patients.” The most serious concerns relate to potential iatrogenic effects: Could telling someone that he or she is at a high statistical risk for suicide increase hopelessness? Other concerns relate to perceived violations of privacy, negative effects on the therapeutic relationship, and interventions that reduce patient engagement in treatment or that are viewed as unhelpful or uncaring.
Investigators from several groups, including the U.S. Army (12) and Kaiser Permanente (13), are considering advanced statistical procedures to improve suicide prevention programs. The U.S. Department of Veterans Affairs (VA) has already implemented a predictive program for suicide prevention: Recovery Engagement and Coordination for Health–Veterans Enhanced Treatment (REACH VET). The program uses a predictive model that analyzes patients’ electronic health records once a month to identify veterans with a high statistical risk for adverse outcomes, with a focus on suicide. An initial development and suicide mortality validation study was conducted (14), followed by retesting and a comparison of the potential benefits of a variety of machine-learning approaches (15).
The final statistical model utilizes a penalized logistic regression model. Following identification, a series of clinical and prevention interventions are considered for approximately 6,000 veterans, nationwide, each month (14). A local clinician who has worked with the veteran is prompted to review the treatment plan (e.g., frequency of mental health appointments, use of evidence-based treatments, reevaluation of pharmacotherapy) and to consider treatment enhancements that might reduce risk for suicide (e.g., safety plan, increased monitoring of stressful life events, telehealth, and the Caring Letters intervention) (16).
The intervention also requires clinicians to reach out to veterans to offer support, assess risk, and collaboratively consider treatment modifications. This requirement may represent a challenging step for clinicians. At one VA facility, providers documented consideration of additional prevention services for 100% of more than 300 identified REACH VET patients, and a large majority of providers conducted outreach to veterans as part of their review of treatment (16). However, only 7% of providers informed the veteran that he or she was identified as being at a high statistical risk for suicide or other adverse outcomes (16). Among the several plausible interpretations of these findings, it is possible that clinicians are concerned about how patients will react to such discussions. In fact, on the basis of feedback from the field, the national REACH VET program developed resources to assist clinicians in conversations with patients (e.g., a vignette script describing what a provider could say to a patient or video training sessions) (17).
To our knowledge, no study has collected information from patients about their perspectives on the use of predictive models for suicide prevention. In this study, we aimed to collect feedback from high-risk veterans who were hospitalized in a psychiatric inpatient unit.
Methods
Participants
Psychiatric inpatients were consecutively recruited at the inpatient unit of a large VA health care facility. Participants were recruited because they were at high risk for being identified by REACH VET given that psychiatric inpatient treatment is considered in the predictive model. Patients were excluded if they had severe dementia, mania, psychosis, or cognitive impairment or disorientation that rendered the individual unable to complete the survey. An attempt was made to invite all appropriate patients to complete the anonymous survey; 134 veterans were excluded. Out of 220 patients approached about the study, 32 declined to participate. Surveys were distributed to 188 patients, and 102 surveys were returned (54%).
Survey
The survey included a form to collect demographic characteristics and three different vignettes that described an initial conversation that a clinician might have with a patient to introduce a suicide prevention program on the basis of predictive analytics (Box 1). The VA’s preliminary script that is used to help VA clinicians discuss the REACH VET program with veterans was included (script A). Given the false-positive problem inherent in any predictive model for suicide (8), the second vignette described prediction results as a “screening” and provided more statistical information (script B). The third vignette was intended to represent a more collaborative approach with a patient (script C).
BOX 1. Vignettes introducing a U.S. Department of Veterans Affairs (VA) suicide prevention program to patients
Script A
Hi [veteran’s name]. I am calling to let you know about a new program called REACH VET that we have here in the VA and to check in to see how you are doing. REACH VET uses information about risk factors that are in a veteran’s medical record to identify those who are at risk for things like hospitalizations and death caused by illness or suicide. Risk factors in your medical record tell us that you are at elevated risk for these things, so I wanted to check in with you to see how you are doing and to see how I can help best meet your needs. I also took a look at your chart and thought about some additional ways we can be supporting you, and I would like to share those if it’s alright with you.
Script B
Hi [veteran’s name]. I am calling to say hi and to see if I could set a time to talk to you about some screening results we obtained. Do you have time to talk now?
[If yes]
The VA routinely reviews medical records to try to identify veterans we might be able to better assist. The program statistically analyzes the information to try to find veterans who might be at a higher risk for things like hospitalization or life‐threatening illnesses or even suicide. We do not know if the results actually apply to you. In fact, in the vast majority of cases, they do not apply to the people who the statistical model identifies. But this is important, so we like to check in with veterans. Your name was identified by the program, so I wanted to check in with you to see how you are doing and assess what this might mean for you. We can also discuss how I can help to best meet your needs.
Script C
Hi [veteran’s name]. I’m calling to let you know about a new program called REACH VET that we have here in the VA and to check in to see how you are doing. REACH VET uses information about risk factors that are in a veteran’s medical record, such as diagnoses and medications, to identify those who may be at higher risk for things like hospitalizations and death caused by illness or suicide. Risk factors in your medical record suggest that you may be at elevated risk for these things. Specifically, the factors identified in your chart include [insert risk factors that contributed to the veteran’s identification by REACH VET]. I was alerted that you had been identified by the REACH VET program, so I wanted to check in with you to see how you are doing and to see how I can help best meet your needs. First, do you have any questions so far?
[If none]
I was hoping to talk with you about possible steps we can take to better support your needs. Do you have any ideas for ways in which we could help lower your risk? I looked at your chart and thought about some additional ways we can support you. Do you mind if I share those with you?
The order of the vignette presentations was counterbalanced, and each vignette was followed by five items (rated on a 5-point Likert scale) that requested feedback about that vignette (i.e., ratings of caring vs. uncaring, helpful vs. unhelpful, informative vs. uninformative, encouraging vs. discouraging, and how well participants understood the program). Patients were also asked what they liked and did not like about each vignette (free response). Then, each respondent was asked which vignette they preferred most. They rated that vignette (their most preferred) on six items (rated on a 5-point Likert scale) related to therapeutic alliance, treatment engagement, privacy, and hopelessness. A free response question also asked, “What advice would you give to providers when contacting a veteran selected through REACH VET?”
Procedure
Anonymous surveys were provided to appropriate patients during their stay at the psychiatric inpatient unit. Although these patients were at high risk for suicide on the basis of their clinical characteristics, their identification status in REACH VET was unknown (although psychiatric inpatient stays are included in the REACH VET predictive model). The survey packet included an information sheet describing the purpose and intent of the survey as well as how to complete and return it. To maintain anonymity, we obtained informed consent by using an information sheet procedure that did not require a participant signature and that was approved by our local institutional review board.
Data Analysis
We compared ratings by vignette type by using the Friedman test. Dunn pairwise post hoc tests were conducted when indicated with a Bonferroni adjustment for multiple tests. All percentages are based on valid responses.
Results
Ratings by Vignette
Table 1 describes the demographic characteristics of the sample (Ns for each analysis vary on the basis of missing data). All three vignettes were rated as neutral to very caring by more than 80% of respondents (with at least 69% of respondents rating all vignettes as somewhat caring to very caring) (Figure 1). There was a relative difference in ratings. For example, script C was rated as neutral to very caring by 95% (N=88) of respondents. The overall difference in vignette ratings was significant (χ2=7.16, df=2, p=0.03), but post hoc comparisons were not significant.
Characteristic | N | % |
---|---|---|
Sex | ||
Male | 73 | 85 |
Female | 11 | 13 |
Other | 2 | 2 |
Age | ||
17–19 | 0 | 0 |
20–24 | 1 | 1 |
25–29 | 7 | 8 |
30–34 | 12 | 14 |
35–39 | 8 | 9 |
40–44 | 11 | 13 |
45–49 | 8 | 9 |
50–54 | 13 | 15 |
55–59 | 5 | 6 |
60–64 | 11 | 13 |
65–69 | 7 | 8 |
≥70 | 3 | 4 |
Race | ||
Caucasian | 70 | 82 |
African American | 12 | 14 |
American Indian or Alaska Native | 2 | 2 |
Native Hawaiian or Pacific Islander | 0 | 0 |
Asian | 1 | 1 |
Biracial, multiracial, or other | 6 | 7 |
Ethnicity | ||
Non-Hispanic | 67 | 92 |
Hispanic | 6 | 8 |
Education level | ||
Some high school | 4 | 5 |
Alternative high school certificate | 2 | 2 |
High school graduate | 5 | 6 |
Some college, no degree | 37 | 44 |
Associate’s degree or technical certificate | 20 | 24 |
4-year college degree | 10 | 12 |
≥Master’s degree | 7 | 8 |
Marital status | ||
Never married | 20 | 24 |
Married | 20 | 24 |
Legally separated | 7 | 8 |
Divorced | 35 | 42 |
Widowed | 2 | 2 |
Military rank or grade at discharge | ||
Cadet or midshipman | 0 | 0 |
E1–E4 | 57 | 67 |
E5–E9 | 23 | 27 |
Unknown enlisted | 1 | 1 |
Officer | 4 | 5 |
Warrant officer | 0 | 0 |
War era during serviceb | ||
World War II | 0 | 0 |
Korean conflict | 0 | 0 |
Vietnam era | 16 | 19 |
Gulf War | 22 | 27 |
OEF or OIFc | 14 | 16 |
Peacetime | 19 | 22 |
Other | 14 | 16 |
Combat exposure | 36 | 44 |
TABLE 1. Demographic characteristics of veterans in a psychiatric inpatient unita
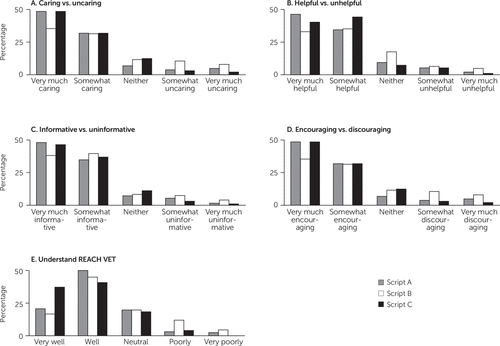
FIGURE 1. Ratings from veterans in a psychiatric inpatient unit, by vignette introducing a suicide prevention programa
aREACH VET, Recovery Engagement and Coordination for Health–Veterans Enhanced Treatment.
A similar pattern emerged for ratings of helpfulness. All vignettes were rated as neutral to very helpful by nearly 90% of respondents (with at least 70% of respondents rating them all as somewhat helpful to very helpful). Again, there was a difference in ratings noted in the omnibus test (χ2=9.49, df=2, p=0.009). Script A was rated higher than script B (p=0.03), but comparisons were no longer statistically significant after adjustment by the Bonferroni correction (p=0.08).
All three vignettes were rated as neutral to very informative by at least 88% of respondents (with at least 79% of respondents rating them all as somewhat informative to very informative). Script C was rated as neutral to very informative by 96% (N=90) of respondents. There was no difference in ratings between vignettes (χ2=5.40, df=2, p=0.07).
All three vignettes were rated as neutral to very encouraging by at least 78% of respondents (with at least 60% of respondents rating them all as somewhat encouraging to very encouraging). Script C was rated as neutral to very encouraging by 89% (N=83) of respondents. There was a significant difference between vignette ratings overall (χ2= 7.92, df=2, p=0.02). Script A was rated higher than script B (p=0.04), but comparisons were no longer statistically significant after adjustment by the Bonferroni correction (p=0.12).
Patients were asked to rate how well they understood the prevention program after reading each vignette. All three vignettes were rated as neutral to very well understood by at least 83% of respondents (with at least 63% of respondents rating them all as well to very well understood). Script C was rated as neutral to very well understood by 97% (N=85) of respondents. There was a significant difference between vignette ratings overall (χ2=11.17, df=2, p=0.004), with post hoc comparisons revealing more favorable ratings for script C compared with script B, even after adjustment by the Bonferroni correction (p=0.039).
Preferred Vignette
Participants were asked to select the vignette that they preferred. Script C was selected more frequently (N=36; 42%) than script A (N=25; 29%) or script B (N=24; 28%), but these differences were not statistically significant (χ2=3.13, df=2, p=0.21).
Qualitative feedback indicated that some veterans appreciated the collaborative elements that were incorporated into script C. When asked why it was their favorite, responses included, “Because it puts it in the hands of the vet,” and “Warmest approach.” One participant noted that it “asks more questions that makes it seem more personal to me and less forced on me.”
Many veterans who preferred script A appreciated the language that was noted to be “short and concise, easy to…understand.” On the other hand, some participants who preferred script B noted its efforts to highlight potential problems with false positives. Comments included, “Would not alarm the vet like the other two,” and “Gentler-balanced.”
Ratings Related to Hopelessness, Privacy, the Clinician, and Treatment Engagement
Feedback was requested from all participants about how they would feel if they were identified by the model and had the discussion from their favorite vignette with their clinician. A large majority of patients (78%) disagreed or strongly disagreed that they would feel hopeless after the discussion (Table 2). Most of the remaining responses were neutral (14%). Only three respondents strongly agreed, and four respondents agreed.
Strongly agree | Agree | Neutral | Disagree | Strongly disagree | ||||||
---|---|---|---|---|---|---|---|---|---|---|
Item | N | % | N | % | N | % | N | % | N | % |
I would feel comfortable discussing my problems with provider | 34 | 37 | 42 | 46 | 10 | 11 | 1 | 1 | 5 | 5 |
I would feel like my provider was “just doing their job”; had no real interest | 4 | 4 | 12 | 13 | 21 | 23 | 40 | 44 | 14 | 15 |
I would feel encouraged to ask questions | 34 | 37 | 39 | 42 | 13 | 14 | 4 | 4 | 2 | 2 |
I would feel involved in treatment plan | 34 | 37 | 37 | 40 | 13 | 14 | 5 | 5 | 3 | 3 |
I would feel my privacy was respected | 35 | 38 | 25 | 27 | 24 | 26 | 5 | 5 | 3 | 3 |
I would feel hopeless after discussion | 3 | 3 | 4 | 4 | 13 | 14 | 29 | 32 | 41 | 46 |
TABLE 2. Ratings from veterans in a psychiatric inpatient unit for their favorite vignette introducing a suicide prevention programa
Similar results were observed for potential privacy concerns. When asked whether they would feel that their privacy was respected, 91% of respondents indicated neutral to strongly agree.
When asked whether they would feel comfortable discussing their problems with the clinician, 83% of respondents agreed or strongly agreed. Slightly more concern was noted when asked whether they would “feel like my provider was ‘just doing their job’ and did not have a real interest in my problems,” as 17% of respondents agreed or strongly agreed.
Two items asked about whether participants would feel involved in treatment and feel comfortable asking questions; 77% of respondents agreed or strongly agreed that they “would feel involved in making decisions about my treatment plans.” Most of the remaining respondents were neutral (14%). When asked whether they “would feel encouraged to ask any questions I may have,” 79% of respondents agreed or strongly agreed.
Qualitative Advice for Clinicians
Veterans were asked what advice they would give to clinicians when contacting patients selected for the prevention program. Overwhelmingly, the advice emphasized a patient-centered approach to care. Veterans encouraged clinicians to be courteous, patient, sincere, respectful, and caring. Example advice included, “Be genuine and compassionate.” Another veteran stated, “Really care.” A third stated, “Be patient and hope with me.” Overall, clinicians were advised to listen, connect on a human level, and avoid overwhelming the patient with information. Of the 70 patients who offered advice, 56% (N=39) included advice related to this theme.
Advice that focused more specifically on the predictive analytics aspect of the prevention program was rarer. However, one veteran said, “Be cautious about creating concern or fear in the vet based on a computer program’s calculations.” Another veteran stated, “I would expect to hear privacy concerns from patients….Why was my record selected, if my file has been flagged, if this will change any treatments I have scheduled.” One veteran provided program implementation advice: “Receiving a call stating the vet is predisposed [or] a possible candidate for an illness is scary and concerning. Maybe have immediate appts open (next day or same week) for the veterans who are contacted.”
Discussion
To our knowledge, this is the first study designed to examine feedback from patients about the use of predictive analytics for suicide prevention. Overall, the results appear to provide early support for the acceptability of using predictive models to identify at-risk patients. After reading the VA’s script that is provided to clinicians nationally as a model to use for conversations with patients, most veterans at high risk for suicide rated the script as caring, helpful, encouraging, and informative. Most patients also reported that they understood the goals of the program after reviewing the brief script. Similar results were obtained for two adaptations of the VA’s script.
Generally, our sample did not endorse some of the most worrisome potential patient concerns. Seventy-eight percent of the patients disagreed or strongly disagreed that they would feel hopeless after a discussion with a health care provider who identified them as high risk on the basis of a predictive model. Almost all other participants’ responses were neutral. Similar results were obtained for other important topics; results suggest that concerns about perceived privacy violations, negative effects on the therapeutic relationship, or negative impacts on treatment engagement may be uncommon. In fact, the results suggest that most patients may feel involved in treatment planning after the discussion.
Although negative reactions appear to be rare (at least in response to our vignettes), it will be important for clinicians and researchers to develop strategies to manage negative patient reactions when they occur. Patient preferences that differ from clinical practice are not uncommon in medicine or mental health care (18). However, predictive models in suicide prevention are unique because they use medical data in a way that the patient may not anticipate. It may be helpful to permit patients to “opt in” to such prevention programs (or at least “opt out”) and to ensure that providers are prepared to use strategies that exist in the literature to help build trust and rapport with patients (19).
There were some indications in the results that script C (the collaborative approach) was more favorably rated than the preliminary VA REACH VET script or the script that described the results in terms of screening. This finding was consistent with the qualitative advice that the respondents wrote for clinicians, in which patients were much more concerned with how clinicians interacted with veterans, in general, than how clinicians described the predictive model results. However, each of the three scripts was preferred as the “favorite” version by at least 28% of the sample. Therefore, although it may be helpful to incorporate some elements of script C in future efforts, it is likely that an ideal prototype would include elements of all three scripts. It is also unlikely that a single script will be able to fully describe the important, complex clinical skills required to anticipate a patient’s communication preferences and to adjust approaches on the basis of patient reactions.
To our knowledge, the VA is the only health care system in the country to implement a suicide prevention program based on a predictive model. Preliminary program information and leadership analyses suggest that the program has been well received by veterans (20). Our study results suggest that the VA’s preliminary vignette to support provider discussions with patients should be well received by most veterans. There are opportunities to consider elements of the other vignettes or to develop a more comprehensive guide for providers on managing these complex conversations.
Because of this study’s limitations, we consider our results to be preliminary. Although the sample included a high-risk cohort, these veterans were not selected on the basis of their identification by the REACH VET statistical model; therefore, results may not generalize to REACH VET patients or to patients enrolled in other health care systems. The views of veterans receiving inpatient treatment might not represent the views of veterans who avoid or refuse mental health care completely. Participants who volunteered may differ in some important ways compared with other inpatients. Veterans were reacting to hypothetical scenarios rather than actual experiences. In addition, although providers might use scripts as a resource to start a conversation with a patient, they do not capture the complex verbal and nonverbal interactions between a patient and provider.
Future research should examine the characteristics of patient feedback sessions (among individuals identified by predictive models) that predict successful outcomes. Patient preferences may not relate to efficacy or effectiveness; just because most veterans seemed to appreciate the vignettes does not indicate that the prevention program is effective. More research is needed on the outcomes of the REACH VET program and other prevention programs based on predictive models. Some comparisons may not have achieved statistical significance because of the sample size. Because there were no existing measures available for the goals of the study, the survey used here was created, and the psychometric properties were unknown.
Conclusions
Most high-risk veterans rated a variety of approaches to discussing results from a predictive model as caring, helpful, encouraging, and informative. Most patient advice for clinicians emphasized the importance of using a respectful, caring, patient-centered approach to discussions. Although these results support the feasibility of using predictive analytics for suicide prevention, this preliminary study represents only a first step; more research is needed to examine a variety of stakeholder opinions utilizing improved methods.
1 : Big data and machine learning in health care. JAMA 2018; 319:1317–1318Crossref, Medline, Google Scholar
2 : Machine learning for targeted display advertising: transfer learning in action. Mach Learn 2014; 95:103–127Crossref, Google Scholar
3 : Machine learning for suicide research—can it improve risk factor identification? JAMA Psychiatry 2019; 77:13–14Crossref, Google Scholar
4 Hedegaard H, Curtin SC, Warner M: Suicide Mortality in the United States, 1999–2017. Data brief no 330. Hyattsville, MD, National Center for Health Statistics, 2018. https://www.cdc.gov/nchs/products/databriefs/db330.htm. Accessed February 3, 2020Google Scholar
5 2019 National Veteran Suicide Prevention Annual Report. Washington, DC, US Department of Veterans Affairs, 2019. https://www.mentalhealth.va.gov/docs/data-sheets/2019/2019_National_Veteran_Suicide_Prevention_Annual_Report_508.pdfGoogle Scholar
6 : Risk factors for suicidal thoughts and behaviors: a meta-analysis of 50 years of research. Psychol Bull 2017; 143:187–232Crossref, Medline, Google Scholar
7 : The use of machine learning in the study of suicidal and non-suicidal self-injurious thoughts and behavior: a systematic review. J Affect Disord 2019; 245:869–884Crossref, Medline, Google Scholar
8 : Prediction models for suicide attempts and deaths: a systematic review and simulation. JAMA Psychiatry 2019; 76:642–651Crossref, Medline, Google Scholar
9 : Suicide prediction models: a critical review of recent research with recommendations for the way forward. Mol Psychiatry 2020; 25:168–179Crossref, Medline, Google Scholar
10 : Positive predictive values and potential success of suicide prediction models. JAMA Psychiatry 2019; 76:868–869Crossref, Medline, Google Scholar
11 : Ethical and practical considerations in the use of a predictive model to trigger suicide prevention interventions in healthcare settings. Suicide Life Threat Behav 2019; 49:382–392Crossref, Medline, Google Scholar
12 : Predicting suicides after psychiatric hospitalization in US Army soldiers: the Army Study to Assess Risk and Resilience in Servicemembers (Army STARRS). JAMA Psychiatry 2015; 72:49–57Crossref, Medline, Google Scholar
13 : Predicting suicide attempts and suicide deaths following outpatient visits using electronic health records. Am J Psychiatry 2018; 175:951–960Link, Google Scholar
14 : Predictive modeling and concentration of the risk of suicide: implications for preventive interventions in the US Department of Veterans Affairs. Am J Public Health 2015; 105:1935–1942Crossref, Medline, Google Scholar
15 : Developing a practical suicide risk prediction model for targeting high-risk patients in the Veterans Health Administration. Int J Methods Psychiatr Res 2017; 26:
16 : Integrating predictive modeling into mental health care: an example in suicide prevention. Psychiatr Serv 2019; 70:71–74Link, Google Scholar
17 Health Administration’s REACH VET Predictive Modeling and Clinical Program. Paper presented at the International Academy of Suicide Research-American Foundation for Suicide Prevention International Summit on Suicide Research, Miami, 2019Google Scholar
18 : Treatment preferences of psychotherapy patients with chronic PTSD. J Clin Psychiatry 2016; 77:363–370Crossref, Medline, Google Scholar
19 : Building trust and rapport early in the new doctor-patient relationship: a longitudinal qualitative study. BMC Med Educ 2017; 17:32Crossref, Medline, Google Scholar
20 : It Shouldn’t Be This Hard to Serve Your Country: Our Broken Government and the Plight of Veterans. New York, PublicAffairs, 2019Google Scholar