User Engagement in Mental Health Apps: A Review of Measurement, Reporting, and Validity
Abstract
Objective:
Despite the potential benefits of mobile mental health apps, real-world results indicate engagement issues because of low uptake and sustained use. This review examined how studies have measured and reported on user engagement indicators (UEIs) for mental health apps.
Methods:
A systematic review of multiple databases was performed in July 2018 for studies of mental health apps for depression, bipolar disorder, schizophrenia, and anxiety that reported on UEIs, namely usability, user satisfaction, acceptability, and feasibility. The subjective and objective criteria used to assess UEIs, among other data, were extracted from each study.
Results:
Of 925 results, 40 studies were eligible. Every study reported positive results for the usability, satisfaction, acceptability, or feasibility of the app. Of the 40 studies, 36 (90%) employed 371 indistinct subjective criteria that were assessed with surveys, interviews, or both, and 23 studies used custom subjective scales, rather than preexisting standardized assessment tools. A total of 25 studies (63%) used objective criteria—with 71 indistinct measures. No two studies used the same combination of subjective or objective criteria to assess UEIs of the app.
Conclusions:
The high heterogeneity and use of custom criteria to assess mental health apps in terms of usability, user satisfaction, acceptability, or feasibility present a challenge for understanding real-world low uptake of these apps. Every study reviewed claimed that UEIs for the app were rated highly, which suggests a need for the field to focus on engagement by creating reporting standards and more carefully considering claims.
HIGHLIGHTS
In response to the low uptake and low sustained use of mobile mental health apps, this systematic review examined studies evaluating mental health apps for depression, bipolar disorder, schizophrenia, and anxiety.
Of the 40 studies reviewed, 36 used at least some subjective criteria (371 subjective questions) and 25 used at least some objective criteria (71 measures) to assess user engagement.
Every study concluded that the user engagement indicators for the app were positive; however, no study used the same combination of criteria or the same thresholds to evaluate the app.
Mobile technologies are increasingly owned and utilized by people around the world. With this rise in pervasiveness comes the potential to increase access to and augment delivery of mental health care. This can occur in multiple ways, including patient-provider communication, self-management, diagnosis, and even treatment (1). Early evidence concerning the efficacy of mobile mental health apps has created a wave of enthusiasm and support (2). The potential scalability of these app-based interventions has been proposed as a means of addressing the global burden of mental illnesses and offering services to those who are in need but previously have not been able to access care (3). Even in developed countries, where access to mental health services remains inadequate, app-based interventions have been proposed as innovative research, screening, prevention, and care delivery platforms (4). The 10,000 mental health apps currently available for immediate download from the Apple iTunes or Google Android Play marketplaces speak to their easy availability, as well as to the high interest (5).
But potential, interest, or availability alone has not translated into the often-forecasted digital revolution for mental health. Many possible explanations exist, and one factor is the poor uptake of mental health apps (6). User engagement studies can shed valuable insight here. Many studies that evaluate mental health apps include an examination of usability, user satisfaction, acceptability, or feasibility. These “user engagement indicators” (UEIs) are meant to represent the ability of an app to engage and sustain user interactions. However, the lack of guidelines, consensus, or specificity regarding user engagement in mental health research introduces the concerning potential for UEIs to be selected inappropriately, presented with bias, or interpreted incorrectly. Thus it is difficult to interpret, let alone compare or pool data on, engagement metrics related to these smartphone apps. For example, in one study, participants described an app as “buggy,” “clunky,” and “didn’t really work” during qualitative interviews (7). Nevertheless, when the same participants were asked specifically whether the app was “user friendly” and “easy to use,” five of seven reported that the app was user friendly and easy to use. The authors used the responses to the second set of metrics as the basis for their conclusion that the app had positive UEIs, which masked potentially serious usability and safety concerns.
To both assess the current state of reporting and inform future efforts, we performed a systematic review of how the UEIs of apps designed for persons with depression, bipolar disorder, schizophrenia, and anxiety are evaluated. We hypothesized that there would be conflations in the definitions and criteria for common types of UEIs (namely, usability, satisfaction, acceptability, and feasibility), inconsistent subjective and objective criteria used to evaluate UEIs, and inconsistent thresholds of UEI ratings across studies.
Methods
Search String and Selection Criteria
We conducted a systematic search of PsycINFO, Ovid MEDLINE, the Cochrane Central Register of Controlled Trials and the AMED, Embase and HMIC databases on July 14, 2018, using terms synonymous with mobile apps for mental health. The full search algorithm is presented in Table 1. Inclusion criteria were as follows: report original qualitative or quantitative data; primarily involve a mobile application; be designed for people with depression, bipolar disorder, schizophrenia, or anxiety (including posttraumatic stress disorder and obsessive-compulsive disorder); include a conclusion about UEIs for the app (including usability, satisfaction, acceptability, or feasibility); and have a study length of at least 7 days. Reviews, conference reports, protocols, or dissertations were excluded, as were non-English language publications and publications that did not focus on the technologies or diseases of interest. All publications were screened by two authors (MMN and JT), and any disagreements were resolved through discussion resulting in consensus.
Category | Search wordsb |
---|---|
Population | Depression, depressive, mental illness, mental health, mood disorder, affective disorder, anxiety, phobia, bipolar, psychosis, schizophr* |
Intervention | Smartphone*, smart phone*, mhealth, mobile phone*, iphone*, android, mhealth, mobile app*, phone app* |
Comparator | [any] |
Outcome | Usability, user interface, ui, feasib*, pilot, engag*, acceptability |
TABLE 1. Search algorithm for studies of mobile mental health apps, by PICO framework categorya
Data Extraction and Synthesis
A tool was developed to systematically extract data, and the following data were gathered by two authors (MMN and JT). Study details included the study design (e.g., single arm or randomized controlled trial), sample size, inclusion criteria, and clinical characteristics of participants. Intervention details included information about the app, length of the intervention, and device type used. Data on objective UEIs included usage frequency, response to prompts, and trial retention. Data on subjective UEIs included satisfaction questionnaires, interviews about usability, and other similar data. Data were also gathered on factors that might influence usability, such as whether patients were involved in the app design process, incentives for participation, and other similar factors. Institutional review board approval was not required for this literature review.
Results
Included Studies
The initial database search returned 925 results. (A PRISMA chart in an online supplement to this article shows the full study selection process.) The 925 articles were reduced to 882 after duplicates were removed. A further 778 articles were excluded after the titles and abstracts were reviewed for eligibility. Full-text versions were retrieved for 104 articles, of which 64 were ineligible for various reasons (see PRISMA chart in online supplement).
Thus a total of 40 studies reporting UEIs of mental health apps for persons with mental illness were included (7–47). Of these, nine apps were designed for individuals with depression (11, 12, 19, 21, 27, 29, 40, 43, 46), four for those with bipolar disorder (16, 20, 22, 47), seven for those with schizophrenia (23, 25, 28, 33, 38, 39, 45), and seven for those with anxiety (15, 26, 30–32, 35, 44). Thirteen apps were designed for two or more populations with different mental illnesses (8–10, 13, 14, 17, 18, 24, 34, 36, 37, 41, 42)
The mean number of participants enrolled was 32 per study (range two to 163). Of studies that reported the length or mean length of the study (some studies lasted as long as participants wanted to use the app), the mean length was 58 days.
UEIs: Usability, Satisfaction, Acceptability, and Feasibility
Every study performed an evaluation of the usability, satisfaction, acceptability, or feasibility of an app. Although we refer to these criteria as UEIs, the studies reviewed did not use UEI as a term or a framework. Across studies, conflations were noted in the definitions of and criteria for usability, satisfaction, acceptability, or feasibility. Some studies referred to these types of UEI interchangeably. For example, multiple studies used the phrase “usability/acceptability” (23, 24) (and another used the phrase “acceptability/usability” [25]). One referred to a “satisfaction/usability interview” (44). Another study first used the phrase “tolerability and usability” and later switched to “acceptability and tolerability” (47). Another first noted that “acceptability was measured by examining self-reports and user engagement with the program” but later stated that “acceptability was measured by examining users’ self-reported attitudes and satisfaction” (43). Yet another study used the technology acceptance model to partly evaluate the usability of an app (44).
Some studies treated certain UEIs as determinants of others. One study stated, “The BeyondNow app was also shown to be feasible given the high level of usability” (36). Another noted, “To evaluate acceptability of using a smartphone application as part of EP [early psychosis] outpatient care, participants completed self-report surveys at the end of the study evaluating satisfaction” (14). And under the subheading “Aim I–Feasibility: Mobile App Satisfaction,” another study reported, “Participants provided high usability ratings for the mobile app based on the SUS [System Usability Scale]” (8).
Most studies evaluated multiple UEIs at once. Eight drew conclusions about one type of UEI (e.g., usability only) (10, 12, 18, 21, 27, 30, 37, 41), 11 about two types of UEI (e.g., feasibility and acceptability) (9, 17, 19, 29, 31, 36, 38, 40, 44, 46, 47), 11 about three types (11, 14–16, 20, 23, 24, 28, 34, 39, 45), and 10 about four types (8, 13, 22, 25, 26, 32, 33, 35, 42, 43). Furthermore, most studies used the same criteria to evaluate multiple UEIs. For instance, one stated, “Satisfaction, usability and acceptability were calculated based on the percentage of answers of the Likert-scale” (22). The fact that most studies used similar methods to evaluate more than one type of UEI speaks to the lack of precision and distinction between evaluation methods.
Types of Criteria: Subjective and Objective
The criteria used to draw conclusions about UEIs varied widely across studies, as shown in Figure 1. Of the 40 studies reviewed, 15 (38%) concluded that the app had positive UEIs entirely on the basis of subjective criteria (10, 12, 15, 18, 23, 27, 29, 30–32, 37, 41, 44, 46, 47). Four (10%) concluded that the app had positive UEIs entirely on the basis of objective criteria (9, 17, 21, 28), and 21 (53%) concluded that the app had positive UEIs on the basis of a combination of subjective and objective criteria (8, 11, 13, 14, 16, 19, 20, 22, 24–26, 33–36, 38, 39, 40, 42, 43, 45).
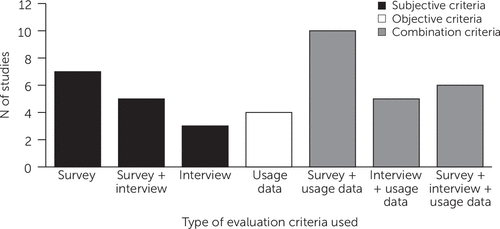
FIGURE 1. Types of criteria used to evaluate user engagement indicators in 40 studies of mental health apps
Subjective criteria
The 36 studies (90%) that evaluated UEIs entirely or partially on the basis of subjective criteria relied on 371 indistinct questions (see table in online supplement) and were assessed by using surveys, interviews, or both. As shown in Table 2, a total of 13 studies derived inspiration from one or more preexisting assessment tools (48–58). The remaining 23 studies did not rely on preexisting tools to evaluate subjective criteria, suggesting that they developed their own custom questions. This assortment of both subjective criteria and methodologies for evaluating UEIs demonstrates that there is no gold standard.
Tool | Study |
---|---|
System Usability Scale (48) | Levin et al., 2017 (8); Ben-Zeev et al., 2014 (25); Bauer et al., 2018 (41); Birney et al., 2016 (46) |
Client Satisfaction Questionnaire (49) | Possemato et al., 2017 (15); Wenze et al., 2016 (16); Boisseau et al., 2017 (32) |
Credibility and Expectancy Scale (50) | Wenze et al., 2016 (16); Watts et al., 2013 (27); Boisseau et al., 2017 (32) |
Usefulness, Satisfaction, and Ease Questionnaire (51) | Ben-Zeev et al., 2014 (25); Corden et al., 2016 (40) |
Adaptation of another study's assessment tool (25, 52) | Ben-Zeev et al., 2016 (23); Cernvall et al., 2018 (30) |
Post-Study System Usability Questionnaire (53) | Ben-Zeev et al., 2014 (25) |
Technology Acceptance Model (54) | Price et al., 2017 (44) |
Technology Assessment Model Measurement Scale (55) | Ben-Zeev et al., 2014 (25) |
Therapeutic Alliance Scales for Children–Revised (56) | Pramana et al., 2014 (26) |
Client Evaluation of Services Questionnaire (57) | Pramana et al., 2014 (26) |
Computer System Usability Questionnaire (58) | Pramana et al., 2014 (26) |
TABLE 2. Preexisting assessment tools used to evaluate user engagement indicators in studies of mental health appsa
Objective criteria.
The 25 studies (63%) that evaluated UEIs entirely or partially on the basis of objective criteria relied on 71 indistinct measures of usage data (see online supplement). Of these 25 studies, five set a target usage goal in advance (8, 28, 34, 38, 39) and 20 considered usage data retrospectively (9, 11, 13, 14, 16, 17, 19–22, 24–26, 33, 35, 36, 40, 42, 43, 45) to determine positive UEIs.
Across all studies, a wide array of objective criteria was taken into account, including “average number of peer and coach interactions” (11), “length of time in clinic at enrollment” (14), “(reliable) logging of location” (19), “(number of) active users” (22) and “percentage of participants who were able to use both system-initiated (i.e., in response to prompts) and participant-initiated (i.e., on-demand) videos independently and in their own environments for a minimum of 3 days after receiving the smartphone” (33).
Thresholds of UEIs
All 40 studies concluded that their app had positive UEIs. However, the studies came to the same conclusion in different ways: they evaluated various types of UEIs with different methodologies—from the criteria used (such as subjective ratings and objective data) to the means of assessment (such as a survey, interview, or usage data). In other words, inconsistencies in the UEI evaluation process cast doubt on the studies’ ability to claim that their app was usable, satisfactory, acceptable, or feasible.
Subjective criteria.
Because of the range of both subjective criteria and their evaluation methods, it is impossible to compare the ratings of UEIs across studies. However, it is clear that studies utilized different thresholds for concluding that their app had positive UEIs. For example, of studies that evaluated the subjective criterion “ease of use,” the percentage of users reportedly satisfied with ease of use ranged from 60% (18) to 100% (13). Similarly, the satisfaction scores for ease of use ranged from 79.7% (46) to 92.6% (16). Despite the range of perceptions about the ease of use of an app, every study concluded that its app had positive UEIs.
Objective criteria.
Differences were noted across studies in objective criteria, such as target usage goals and frequency of usage. For example, of studies that set a target usage goal pertaining to task completion, two studies sought completion of over 33% of prompted tasks (28, 39) and another study sought completion of over 70% of prompted tasks (38). Despite this variability, all the studies that set a target usage goal concluded that the apps had positive UEIs on the basis of the usage data. Similarly, studies that considered frequency of usage as an objective criterion reported frequencies ranging from once per day (8) to once every other day (45) and an average of 5.64 times per participant over the course of 2 months (36). Yet each of these trials concluded that its app had positive UEIs.
Discrepancies between thresholds.
Even when an app seems to meet the threshold for positive UEIs on the basis of subjective criteria, it might not meet the threshold on the basis of objective criteria. One study raised the issue of possible discrepancies arising from evaluating UEIs solely on the basis of subjective versus objective criteria: “Analysis of objective use data for another study utilizing PTSD Coach indicates that although app users report positive feedback on usability and positive impact on symptom distress, only 80% of first-time users reach the home screen and only 37% progress to one of the primary content areas” (15). This is an issue not only within studies but also across studies. For instance, five studies that used retention rate as an objective criterion reported retention rates of 80% (35), 83% (11), 91.5% (21), 100% (38), and 100% (45). Yet studies that did not rely on retention rate as a criterion had retention rates as low as 35% (13) and 65.7% (27). All of these studies concluded that their apps had positive UEIs.
Discussion
Despite the real-world challenges of mental health app usability, engagement, and usage, all 40 studies included in this review reported that their app had positive UEIs. The positive reports for usability, satisfaction, acceptability, or feasibility were made whether the studies based their claims on either subjective or objective criteria—or a combination of criteria—and the studies unfailingly interpreted the UEI ratings as positive even when there was a wide range of reports and of usage data. These findings suggest that the authors of the studies did not establish a threshold indicating a positive UEI or that such thresholds were quite low. The inconsistency of the methodologies makes it difficult to define user engagement and how to best design for it. Furthermore, it calls into question the practices used to evaluate mental health apps.
The findings of this review indicate the lack of consensus about what constitutes usability, satisfaction, acceptability, and feasibility for mental health apps. This lack of consensus makes it difficult to compare results across studies, hinders understanding of what makes apps engaging for different users, and limits their real-world uptake. A great deal of ambiguity currently characterizes the distinctions between various types of UEI (see online supplement), which reduces the usefulness of these descriptors. There is thus a clear and urgent need to formulate standards for reporting and sharing UEIs so that accurate assessments and informed decisions regarding app research, funding, and clinical use can be made.
It is concerning that 15 of the 40 (38%) studies concluded that their app had positive UEIs without considering objective data (Figure 1). Qualitative data are unquestionably valuable for creating a fuller, more nuanced picture of participants, because their characteristics—such as language, disorder, and age—largely inform their ability to use an app and their unique experience of an app. However, there is also a need for objective measurements that can be reproduced to validate initial results and create a baseline for generalizing results of any single study. Consequently, a combination of both subjective and objective criteria may be most useful for offering insight into user engagement.
All studies concluded that their apps had positive UEIs on the bases of vastly different subjective and objective criteria (see online supplement). Although the thresholds for assessing a UEI as positive must depend on the specific purpose of the app (e.g., one study claimed that the use of a suicide prevention app by a single individual at a critical moment could be adequate [36]), predetermined thresholds for interpreting UEIs are urgently required for any meaningful conclusions to be drawn. Every study reviewed here claimed that its app had positive UEIs, which makes it difficult to understand the current challenges surrounding usability, engagement, and usage and hinders progress in the field.
This review had several limitations. After our search retrieved 925 studies, we reviewed only those from academic sources that focused on depression, bipolar disorder, schizophrenia, and anxiety. This restricted our discussion to how the academic community views engagement, as opposed to other industries, and limited the types of mental health apps we took into account. In addition, we assumed that it would be possible and useful for at least some dimensions of user engagement to be measured and reported consistently across mental health apps. Of course, apps that are developed for different purposes require their own specific criteria for determining whether they are engaging users or not. However, if every study claims that its app has positive UEIs and no studies use the same evaluation methods, as found in this review, it is difficult to understand and improve the real-world low uptake of these apps. Although publication bias may explain some of the results, the need for reporting standards is still clear. With more than 10,000 mental health apps in the commercial marketplaces, few of which have ever been studied or had assessment results published (5), the number of black boxes is immense when it comes to user engagement in mobile mental health apps. Examining different mental health conditions beyond those targeted in this review may have also yielded different results.
Conclusions
The experience of mental illness is personal, and the technology literacy of individuals is variable, meaning that no single scale or measurement will ever perfectly capture all engagement indicators for all people. But the future of the field of mobile mental health apps depends on user engagement, and the lack of clear definitions and standards for UEIs is harmful—not only to the field, where progress is impeded, but also to patients, who may not know which app to trust. This review has confirmed the necessity of generating more clarity regarding UEIs, which can both promote app usage and enable researchers to learn from each other’s work and design better mental health apps.
This challenge is compounded by the need to design specifically for the needs of individuals with mental illness. On the topic of Web site design, one study reported, “Commonly prescribed design models and guidelines produce websites that are poorly suited and confusing to persons with serious mental illnesses” (59). Given that smartphone apps are often more complex and interactive than Web sites, it is reasonable to assume that truly usable apps for mental illnesses may look different from apps designed for the general population. The inconsistencies illustrated in this study raise the possibility that no engagement indicators were designed to take into account the potentially unique cognitive, neurological, or motor needs arising from mental illnesses. For example, schizophrenia can lead to changes in cognition, depression can affect reward learning, and anxiety can affect working memory. Furthermore, it is important to consider how the intersectional identities of individuals with mental illness also shape their engagement with mental health apps. Combining lessons from technology design with knowledge about mental illnesses, such as schizophrenia (60), and applying these lessons to evaluations of UEIs could serve as a useful starting point. Other fields have found solutions, and the popularity of the engineering field–derived System Usability Scale (used in several studies in this review) indicates the potential of simple but well-validated metrics. Convening a representative body of patients, clinicians, designers, and technology makers to propose collaborative measures would be a welcome first step.
1 : The WPA-Lancet Psychiatry Commission on the Future of Psychiatry. Lancet Psychiatry 2017; 4:775–818Crossref, Medline, Google Scholar
2 : The efficacy of smartphone-based mental health interventions for depressive symptoms: a meta-analysis of randomized controlled trials. World Psychiatry 2017; 16:287–298Crossref, Medline, Google Scholar
3 : Digital technology for treating and preventing mental disorders in low-income and middle-income countries: a narrative review of the literature. Lancet Psychiatry 2017; 4:486–500Crossref, Medline, Google Scholar
4 : Review of use and integration of mobile apps into psychiatric treatments. Curr Psychiatry Rep 2017; 19:96Crossref, Medline, Google Scholar
5 : Needed innovation in digital health and smartphone applications for mental health: transparency and trust. JAMA Psychiatry 2017; 74:437–438Crossref, Medline, Google Scholar
6 : Clinical review of user engagement with mental health smartphone apps: evidence, theory and improvements. Evid Based Ment Health 2018; 21:116–119Crossref, Medline, Google Scholar
7 : A qualitative study of a blended therapy using problem solving therapy with a customised smartphone app in men who present to hospital with intentional self-harm. Evid Based Ment Health 2017; 20:118–122Crossref, Medline, Google Scholar
8 : Evaluating an adjunctive mobile app to enhance psychological flexibility in acceptance and commitment therapy. Behav Modif 2017; 41:846–867Crossref, Medline, Google Scholar
9 : Feasibility, acceptability, and preliminary effectiveness of a peer-delivered and technology supported self-management intervention for older adults with serious mental illness. Psychiatr Q 2018; 89:293–305Crossref, Medline, Google Scholar
10 : Momentum: a smartphone application to support shared decision making for people using mental health services. Psychiatr Rehabil J 2016; 39:167–172Crossref, Medline, Google Scholar
11 : The feasibility, acceptability, and outcomes of PRIME-D: a novel mobile intervention treatment for depression. Depress Anxiety 2017; 34:546–554Crossref, Medline, Google Scholar
12 : Development and preliminary feasibility study of a brief behavioral activation mobile application (Behavioral Apptivation) to be used in conjunction with ongoing therapy. Cognit Behav Pract 2018; 25:44–56Crossref, Medline, Google Scholar
13 : Acceptability of mHealth augmentation of collaborative care: a mixed methods pilot study. Gen Hosp Psychiatry 2018; 51:22–29Crossref, Medline, Google Scholar
14 : Enhancing early psychosis treatment using smartphone technology: a longitudinal feasibility and validity study. J Psychiatr Res 2018; 96:239–246Crossref, Medline, Google Scholar
15 : Development and refinement of a clinician intervention to facilitate primary care patient use of the PTSD Coach app. Transl Behav Med 2017; 7:116–126Crossref, Medline, Google Scholar
16 : An open trial of a smartphone-assisted, adjunctive intervention to improve treatment adherence in bipolar disorder. J Psychiatr Pract 2016; 22:492–504Crossref, Medline, Google Scholar
17 : IntelliCare: an eclectic, skills-based app suite for the treatment of depression and anxiety. J Med Internet Res 2017; 19:e10Crossref, Medline, Google Scholar
18 : Optimization of a digital medicine system in psychiatry. J Clin Psychiatry 2016; 77:e1101–e1107Crossref, Medline, Google Scholar
19 : Accompanying depression with FINE: a smartphone-based approach. Stud Health Technol Inform 2016; 228:195–199Medline, Google Scholar
20 : Daily mood monitoring of symptoms using smartphones in bipolar disorder: a pilot study assessing the feasibility of ecological momentary assessment. J Affect Disord 2016; 191:88–93Crossref, Medline, Google Scholar
21 : Smartphone-based ecological momentary assessment for Chinese patients with depression: an exploratory study in Taiwan. Asian J Psychiatr 2016; 23:131–136Crossref, Medline, Google Scholar
22 : Psychoeducation in bipolar disorder with a SIMPLe smartphone application: feasibility, acceptability and satisfaction. J Affect Disord 2016; 200:58–66Crossref, Medline, Google Scholar
23 : Mobile behavioral sensing for outpatients and inpatients with schizophrenia. Psychiatr Serv 2016; 67:558–561Link, Google Scholar
24 : Using smartphone apps to promote psychiatric and physical well-being. Psychiatr Q 2015; 86:505–519Crossref, Medline, Google Scholar
25 : Feasibility, acceptability, and preliminary efficacy of a smartphone intervention for schizophrenia. Schizophr Bull 2014; 40:1244–1253Crossref, Medline, Google Scholar
26 : The SmartCAT: an m-health platform for ecological momentary intervention in child anxiety treatment. Telemed J E Health 2014; 20:419–427Crossref, Medline, Google Scholar
27 : CBT for depression: a pilot RCT comparing mobile phone vs computer. BMC Psychiatry 2013; 13:49Crossref, Medline, Google Scholar
28 : The feasibility and validity of ambulatory self-report of psychotic symptoms using a smartphone software application. BMC Psychiatry 2012; 12:172Crossref, Medline, Google Scholar
29 : Harnessing context sensing to develop a mobile intervention for depression. J Med Internet Res 2011; 13:e55Crossref, Medline, Google Scholar
30 : A pilot study of user satisfaction and perceived helpfulness of the Swedish version of the mobile app PTSD Coach. Eur J Psychotraumatol 2018; 9(suppl 1):1472990Crossref, Medline, Google Scholar
31 : Comparison of Prolonged Exposure (PE) Coach to treatment as usual: a case series with two active duty soldiers. Mil Psychol 2015; 27:287–296Crossref, Google Scholar
32 : App-guided exposure and response prevention for obsessive compulsive disorder: an open pilot trial. Cogn Behav Ther 2017; 46:447–458Crossref, Medline, Google Scholar
33 : Video-based mobile health interventions for people with schizophrenia: bringing the “pocket therapist” to life. Psychiatr Rehabil J 2018; 41:39–45Crossref, Medline, Google Scholar
34 : Feasibility and acceptability of smartphone assessment in older adults with cognitive and emotional difficulties. J Technol Hum Serv 2016; 34:209–223Crossref, Medline, Google Scholar
35 : A preliminary investigation of a relapse prevention mobile application to maintain smoking abstinence among individuals with posttraumatic stress disorder. J Dual Diagn 2017; 13:15–20Crossref, Medline, Google Scholar
36 : Evaluating the feasibility and effectiveness of an Australian safety planning smartphone application: a pilot study within a tertiary mental health service. Suicide Life Threat Behav 2018Crossref, Medline, Google Scholar
37 : Mobile health (mHealth) versus clinic-based group intervention for people with serious mental illness: a randomized controlled trial. Psychiatr Serv 2018; 69:978–985Link, Google Scholar
38 : Development and feasibility testing of a smartphone intervention to improve adherence to antipsychotic medications. Clin Schizophr Relat Psychoses (Epub ahead of print, July 25, 2016)Medline, Google Scholar
39 : Actissist: proof-of-concept trial of a theory-driven digital intervention for psychosis. Schizophr Bull 2018; 44:1070–1080Crossref, Medline, Google Scholar
40 : MedLink: a mobile intervention to improve medication adherence and processes of care for treatment of depression in general medicine. Digit Health 2016; 2:2055207616663069Crossref, Google Scholar
41 : Applying the principles for digital development: case study of a smartphone app to support collaborative care for rural patients with posttraumatic stress disorder or bipolar disorder. J Med Internet Res 2018; 20:e10048Crossref, Medline, Google Scholar
42 : A mobile health platform for clinical monitoring in early psychosis: implementation in community-based outpatient early psychosis care. JMIR Ment Health 2018; 5:e15Crossref, Medline, Google Scholar
43 : Digital peer-support platform (7Cups) as an adjunct treatment for women with postpartum depression: feasibility, acceptability, and preliminary efficacy study. JMIR Mhealth Uhealth 2018; 6:e38Crossref, Medline, Google Scholar
44 : Tracking post-trauma psychopathology using mobile applications: a usability study. J Technol Behav Sci 2017; 2:41–48Crossref, Medline, Google Scholar
45 : Feasibility of PRIME: a cognitive neuroscience-informed mobile app intervention to enhance motivated behavior and improve quality of life in recent onset schizophrenia. JMIR Res Protoc 2016; 5:e77Crossref, Medline, Google Scholar
46 : MoodHacker mobile web app with email for adults to self-manage mild-to-moderate depression: randomized controlled trial. JMIR Mhealth Uhealth 2016; 4:e8Crossref, Medline, Google Scholar
47 : Experiences of remote mood and activity monitoring in bipolar disorder: a qualitative study. Eur Psychiatry 2017; 41:115–121Crossref, Medline, Google Scholar
48 : SUS: A Quick and Dirty Usability Scale. Reading, United Kingdom, Redhatch Consulting Ltd, 1996; https://hell.meiert.org/core/pdf/sus.pdfGoogle Scholar
49 : Assessment of client/patient satisfaction: development of a general scale. Eval Program Plann 1979; 2:197–207Crossref, Medline, Google Scholar
50 : Psychometric properties of the credibility/expectancy questionnaire. J Behav Ther Exp Psychiatry 2000; 31:73–86Crossref, Medline, Google Scholar
51 : Measuring Usability With the USE Questionnaire. Fairfax, VA, Society for Technical Communication, Usability and User Experience Special Interest Group, 2001. https://www.researchgate.net/profile/Arnold_Lund/publication/230786746_Measuring_Usability_with_the_USE_Questionnaire/links/56e5a90e08ae98445c21561c/Measuring-Usability-with-the-USE-Questionnaire.pdfGoogle Scholar
52 : Preliminary evaluation of PTSD Coach, a smartphone app for post-traumatic stress symptoms. Mil Med 2014; 179:12–18Crossref, Medline, Google Scholar
53 : Psychometric evaluation of the Post-Study System Usability Questionnaire: the PSSUQ. Proc Hum Factors Ergon Soc Annu Meet 1992; 36:1259–1260Google Scholar
54 : User acceptance of information technology: system characteristics, user perceptions and behavioral impacts. Int J Man Mach Stud 1993; 38:475–487Crossref, Google Scholar
55 : A theoretical extension of the technology acceptance model: four longitudinal field studies. Manage Sci 2000; 46:186–204Crossref, Google Scholar
56 : Alliance and outcome in cognitive-behavioral therapy for adolescent depression. J Clin Child Adolesc Psychol 2008; 37:631–639Crossref, Medline, Google Scholar
57 : Assessment of patient satisfaction: development and refinement of a Service Evaluation Questionnaire. Eval Program Plann 1983; 6:299–313Crossref, Medline, Google Scholar
58 : IBM computer usability satisfaction questionnaires: psychometric evaluation and instructions for use. Int J Hum Comput Interact 1995; 7:57–78Crossref, Google Scholar
59 : Designing websites for persons with cognitive deficits: design and usability of a psychoeducational intervention for persons with severe mental illness. Psychol Serv 2007; 4:202–224Crossref, Medline, Google Scholar
60 : Unlocking design potential: design with people diagnosed with schizophrenia. Inform Health Soc Care 2019; 44:31–47Crossref, Medline, Google Scholar