Injurious Assault Rates on Inpatient Psychiatric Units: Associations With Staffing by Registered Nurses and Other Nursing Personnel
Abstract
Objective:
The study examined associations between staffing levels by registered nurses (RNs) and non-RNs on psychiatric units and the rate of injurious assaults against hospital personnel, the rate of injurious assaults against other patients, and the total rate of injurious assaults.
Methods:
Generalized mixed models were used to analyze 2011–2013 data from 461 adult inpatient psychiatric units in 327 U.S. hospitals. Monthly data were aggregated to the unit level. The analyses considered RN and non-RN hours per patient day (HPPD) as linear and nonlinear predictors of the three assault rates, controlling for hospital ownership, size, and teaching status.
Results:
Both staffing variables had statistically significant linear associations with assault rates. Higher values of HPPD for non-RNs were associated with a higher rate of assaults against hospital personnel (exp[B]=1.23), a higher rate of assaults against patients (exp[B]=1.31), and a higher total rate of injurious assaults (exp[B]=1.21). Higher values of HPPD for RNs were associated with a higher rate of assaults against hospital personnel (exp[B]=1.11) and a lower rate of assaults against patients (exp[B]=.81).
Conclusions:
This is the largest published study to date of the association between psychiatric unit staffing and violence and the first to examine separately assaults against hospital personnel and assaults against patients in relation to staffing. Results confirm and qualify previous findings of a positive staffing-violence association. Injurious assaults against hospital personnel and against patients have different associations with nurse staffing and should be studied as separate phenomena with different causes.
Violence by inpatients in psychiatric settings is a prevalent and much-studied problem (1). Although it is asserted that inadequate nurse staffing levels increase the likelihood of violence, the empirical evidence for this claim is weak. In fact, findings from the largest, most rigorous studies of nurse staffing and inpatient violence suggest that violence is more frequent at higher staffing levels (2). In a shift-level study of 136 English psychiatric units, researchers found that rates of physical aggression were higher when a greater number of qualified nurses were on duty and when a greater number of unqualified nurses were on duty (3). In a U.S. study of 351 units, higher levels of total staffing (including both registered nurses [RNs] and non-RN nursing personnel) were associated with higher assault rates; however, as RNs provided a larger proportion of nursing hours relative to other nursing personnel, assault rates tended to be lower (2).
One question that previous research has not answered is how differences in staffing patterns for RNs and non-RNs are related to violence. Staff-patient interactions, especially involving limit setting, are frequently identified as a trigger of aggression or violence (4). Thus if RNs have more responsibility for enforcing rules and denying requests (5), one might expect that RN staffing levels would have a stronger association with assault rates compared with non-RN staffing levels. Alternatively, units with more violent patient populations may employ more non-RN staff to respond to assaults, in which case violence may be more strongly associated with non-RN staffing levels than with RN staffing levels.
Another unanswered question is whether staffing has different associations with assaults against hospital personnel versus assaults against patients. In a meta-analysis, researchers estimated that 39% of reported incidents of aggression or violence were preceded by staff-patient interactions and that 23% were preceded by patient-patient interactions (4). It is likely that assaults on staff and assaults on patients tend not only to have different causes but also to have different associations with staffing; however, these two types of assaults have not been studied as separate phenomena in previous studies of staffing and violence.
This study examined associations of staffing levels by RNs and non-RNs with the rate of injurious assaults against hospital personnel, the rate of injurious assaults against other patients, and the total rate of injurious assaults, allowing for nonlinear associations and for interaction between RN and non-RN staffing. Because of concerns about underreporting of violence (1), the study focused only on injurious assaults, which are presumably less likely to go unreported. It was hypothesized that both RN and non-RN staffing would be positively associated with all three assault rates—in other words, assault rates would be higher on units with higher levels of either type of staffing.
Methods
Sample and Data
Administrative data on assaults and staffing for 2011–2013 were extracted from the National Database of Nursing Quality Indicators (NDNQI), which operates with oversight from the Human Subjects Committee at the University of Kansas Medical Center. Collection of assaults data began, with committee approval, in 2004. The NDNQI and its data are described more fully elsewhere (6). Participating hospitals have the option of reporting monthly data on nursing unit staffing and, for psychiatric units, assaults by patients. Staffing data include hours worked by four types of direct-care nursing personnel: RNs, licensed practical and licensed vocational nurses (LPNs), mental health technicians (MHTs), and assistive personnel.
Assaults data reported by hospitals include the count of inpatient days and injurious assaults each month and whether a unit is locked. For each injurious assault, hospitals classify each of the most seriously injured victims (up to three) as a patient; visitor; RN; LPN; MHT; assistive personnel; security personnel; physician; social worker, psychologist, or counselor; resident or intern; student; other health care provider; other non–health care employee; or other person. Hospitals can also indicate that no documentation was available for classifying the victims.
The NDNQI classifies psychiatric units on the basis of patient population, including adult, geriatric, child or adolescent, dual diagnosis, and behavioral health (for eating disorders and substance abuse). Hospitals have the option to report a subspecialty for each unit. The NDNQI designates units that primarily serve short-stay psychiatric patients as intensive, units that primarily serve non–short-stay patients as general, and units that serve a combination of short-stay and non–short-stay patients as mixed.
The study sample was limited to adult psychiatric units reporting both staffing and assaults data for at least six months during 2011–2013. The final sample comprised 461 units from 311 general and 16 psychiatric hospitals. Forty-five states and Washington, D.C., were represented. Counts of hospitals by U.S. census division were as follows: New England, N=20 (6%); Middle Atlantic, N=80 (24%); South Atlantic, N=68 (21%); East North Central, N= 35 (11%); East South Central, N=61 (19%); West North Central, N=19 (6%), West South Central, N=22 (7%); Mountain, N=10 (3%); and Pacific, 12 (4%). Two-thirds (N=111, 66%) of hospitals were teaching hospitals, and 200 (61%) had fewer than 300 beds. The hospitals were predominantly nonprofit (N=255, 78%); federal (N=25), nonfederal government (N=30), and for-profit (N=17) hospitals accounted for 8%, 9%, and 5%, respectively, of the sample hospitals.
All but 29 (6%) units in the sample were locked. In terms of subspecialty, 283 (61%) units were classified as mixed, 45 (10%) as general, and 39 (8%) as intensive. The remaining 94 (20%) units had no reported subspecialty. Units reported data for an average of 26.3±10.0 of the 36 study months; 408 (89%) units reported at least 12 months of data. Patients’ demographic characteristics are unknown, given that the NDNQI does not generally collect patient-level data except in the event of a specific adverse event.
Study Variables
The three criterion variables of interest were the rate of injurious assaults against hospital personnel, the rate of injurious assaults against patients, and the total rate of injurious assaults. To compute the first two rates, each assault was classified as an assault against hospital personnel (if only hospital personnel were injured), an assault against patients (if only patients were injured), or “other” assault (all other assaults, including incidents in which both hospital personnel and patients were injured and in which the type of victim or victims was unknown). Monthly counts of assaults and patient days were summed across study months for each unit to yield a studywide count of assaults against hospital personnel, a studywide count of assaults against patients, a studywide count of total injurious assaults (comprising all injurious assaults, including “other” assaults), and a studywide count of patient days. Assault rates were computed as assaults per 1,000 patient days.
The focal predictors were hours per patient day (HPPD) for RNs and non-RNs. Non-RN hours were computed by summing hours for LPNs, MHTs, and assistive personnel. Studywide RN and non-RN hours were computed for each unit by summing across the unit’s monthly RN and non-RN hours. HPPD for RNs and non-RNs was computed by dividing the unit’s studywide count of RN hours and non-RN hours, respectively, by the unit’s studywide count of patient days.
Four hospital and unit characteristics were included as control variables in modeling. These were hospital ownership (federal, nonfederal government, for profit, or not for profit), hospital size (<300 or ≥300 beds), hospital teaching status (teaching or nonteaching), and unit locked status (locked or unlocked). Unit subspecialty (intensive, general, or mixed) was examined in a separate sensitivity analysis described below.
Statistical Modeling
Generalized mixed modeling was used to assess the associations between the assault rates and explanatory variables (7). Negative binomial regression models were chosen for their ability to accommodate count data that may be overdispersed; rates are easily modeled by incorporating an exposure variable (in this case, patient days) (8). Each model included a random hospital intercept term to account for nonindependence among units in the same hospital. Models were fit by maximum likelihood by using the GLIMMIX procedure in SAS, version 9.4. Staffing variable values for three units (11.0 and 17.1 HPPD for RNs and 9.6 HPPD for non-RNs) were identified as outliers and were removed to prevent inordinate influence on model estimates.
The final model for each criterion variable was chosen from eight candidate models. Each candidate model included the four control variables (hospital ownership, hospital size, hospital teaching status, and unit locked status). The simplest candidate model (the “linear model”) included only linear terms for HPPD for RNs and non-RNs (both mean centered) in addition to the control variables. The remaining models covered all combinations of the following three second-order terms: HPPD × HPPD for RNs, HPPD × HPPD for non-RNs, and HPPD for RNs × HPPD for non-RNs; these models also included the linear terms and control variables. Thus, in addition to the six-predictor linear model, there were three seven-predictor models (each with one second-order term), three eight-predictor models (each with two second-order terms), and one nine-predictor model (with all three second-order terms). The second-order models were fit to allow for quadratic associations between staffing variables and assault rates and for interaction between the two staffing variables.
All eight candidate models were fit for each criterion variable; the model with the smallest Bayesian information criterion (BIC) value was selected. The BIC is a likelihood-based measure of fit involving a penalty for each parameter estimated, making it ideal for comparing models of different sizes; parsimonious models tend to be favored (9). To assess model explanatory power, a pseudo-R2 value was computed for each of the three final models by squaring the correlation between the observed assault count and the model-predicted count (10).
Conditional studentized residuals were examined for outliers. One observation in the final model had an outlying residual (value >5) for assaults against patients; excluding this observation did not substantially change the model estimates for the focal predictors, so it was retained. An examination of variance inflation factors (all factors <2.0) and condition indices indicated that multicollinearity among explanatory variables was not a problem.
Sensitivity Analysis
The analysis described above included 94 units without a reported subspecialty. To assess the effect of including these units and ignoring subspecialty as an explanatory variable, the primary analysis was rerun on the smaller sample of 367 units with known subspecialty, this time with subspecialty included as a categorical predictor in all models. The model comparison process yielded the same three final models. For all three, results for the focal predictors were comparable to those obtained in the primary analysis, and subspecialty was not statistically significant (with significance set at α=.05).
Results
Units reported over 6.7 million patient days during the study, or an average of 558 patient days per unit per month. There were 5,301 injurious assaults reported, of which 3,687 (70%) were classified as assaults against hospital personnel and 1,299 (25%) as assaults against patients. Overall rates of assaults against hospital personnel, assaults against patients, and total injurious assaults were, respectively, .55, .19, and .78 assaults per 1,000 patient days.
HPPD for RNs on units ranged from .9 to 8.7 (mean±SD=4.2±1.3). Staffing levels for non-RNs were lower, ranging from .0 to 7.4 (mean=3.3±1.3). The number of patients per RN on the unit (computed by dividing 24 by the unit’s HPPD for RNs) ranged from 2.8 to 25.6 (mean=6.4±2.3). When hours provided by non-RNs were included, the number of patients per nurse (computed by dividing 24 by total nursing HPPD) ranged from 1.8 to 9.0 (mean=3.4±.8).
On the basis of the BIC, the linear model was selected for all three criterion variables. Model estimates for the focal predictors are shown in Table 1. There was a positive, statistically significant association between HPPD for non-RNs and the rate of assaults against hospital personnel (exp[B]=1.23), the rate of assaults against patients (exp[B]=1.31), and the rate of total injurious assaults (exp[B]=1.21). In terms of number of patients per nurse, an increase of one HPPD for non-RNs would be equivalent to changing the number of patients per nurse from eight to six.
Type of assault and predictor | B | SE(B) | Exp(B) | 95% CI for exp(B) | p | Pseudo-R2 |
---|---|---|---|---|---|---|
Against hospital personnel | .72 | |||||
HPPD for RNs | .10 | .04 | 1.11 | 1.02–1.21 | .015 | |
HPPD for non-RNs | .21 | .04 | 1.23 | 1.13–1.34 | <.001 | |
Against patients | .53 | |||||
HPPD for RNs | –.20 | .07 | .81 | .71–.93 | .004 | |
HPPD for non-RNs | .27 | .07 | 1.31 | 1.15–1.50 | <.001 | |
Total | .57 | |||||
HPPD for RNs | .02 | .04 | 1.02 | .94–1.10 | .602 | |
HPPD for non-RNs | .19 | .04 | 1.21 | 1.12–1.31 | <.001 |
TABLE 1. Associations between rates of assault and hours per patient day (HPPD) among registered nurses (RNs) and non-RNs on 461 psychiatric units
Higher staffing levels for RNs were associated with higher rates of assaults against hospital personnel (exp[B]=1.11) but lower rates of assaults against patients (exp[B]=.81). Not surprisingly, given these opposite effects, HPPD for RNs was not a statistically significant predictor in the model for total injurious assaults.
Figures 1 and 2 depict model-predicted rates of assaults against hospital personnel and assaults against patients in a hypothetical locked unit in a small, nonprofit teaching hospital. The rates of assault are plotted against HPPD for non-RNs for various levels of RN staffing (fifth, 50th, and 95th percentiles of HPPD for RNs). The rates were computed by choosing the appropriate values for the explanatory variables in the fitted models.
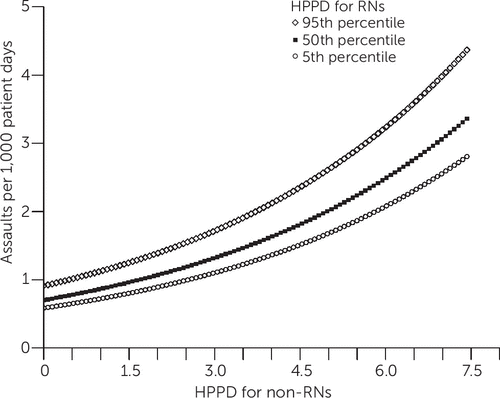
FIGURE 1. Predicted rate of injurious assaults against hospital personnel by HPPD for non-RNs for a hypothetical psychiatric unit at the fifth, 50th, and 95th percentiles for HPPD among RNsa
a HPPD, hours per patient day; non-RNs, nonregistered nurses; RNs, registered nurses
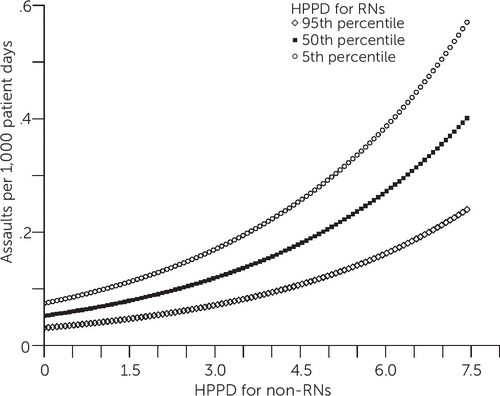
FIGURE 2. Predicted rate of injurious assaults against patients by HPPD for non-RNs for a hypothetical psychiatric unit at the fifth, 50th, and 95th percentiles for HPPD for RNsa
a HPPD, hours per patient day; non-RNs, nonregistered nurses; RNs, registered nurses
Discussion
This is the largest published study to date of staffing levels and assault rates on psychiatric units and the first to conduct separate examinations of the associations between staffing and rates of injurious assaults against hospital personnel and against patients. When all injurious assaults were considered together, units with higher levels of staffing by non-RNs tended to have higher assault rates. This is consistent with results of a previous study in which levels of total nurse staffing (including RN and non-RN hours) were positively associated with the rate of injurious assaults and the rate of total assaults (including noninjurious assaults), whereas the percentage of nursing care hours provided by RNs was inversely associated with both rates (2).
When injurious assaults against hospital personnel and injurious assaults against patients were considered separately, a more complete and interesting picture emerged. There was no evidence that units with higher nurse staffing levels are safer for hospital personnel. On the contrary, HPPD for both RNs and non-RNs had statistically significant positive associations with the rate of injurious assaults against hospital personnel. In terms of assaults against patients, HPPD for RNs and non-RNs had opposite effects: higher levels of non-RN staffing were associated with higher assault rates, but higher levels of RN staffing were associated with lower assault rates.
Various explanations for a positive association between staffing and violence have been offered, but it is difficult to validate any of them, or even to establish the direction of causation, with results from observational studies. These explanations and the issue of causation are discussed in more detail elsewhere (2). Briefly, higher staffing levels may lead to higher assault rates (for example, by increasing patient-staff interaction and conflict), or higher assault rates may lead to higher staffing levels. In a longitudinal study of whether staffing increases precede conflict and containment incidents or vice versa, researchers reported that higher levels of qualified staffing on a shift were associated with higher conflict and containment rates during that shift and subsequent shifts. However, they found little evidence that higher conflict and containment rates on a shift were associated with higher levels of staffing during subsequent shifts (5). Of course, in the longer term, units experiencing a sustained rise in assault rates may increase staffing in an effort to prevent assaults or enhance capacity to respond to assaults. Regardless of the direction of causation, this study’s findings add to the empirical evidence casting doubt on the conventional wisdom that higher staffing levels generally lead to less violence toward hospital personnel (2,3,5,11).
The finding that higher levels of RN staffing were associated with lower rates of assaults against patients is particularly interesting and, given some prior research, unexpected (3). If RN staffing has a causal effect on the rate of assaults against patients, it may be that RNs are particularly adept at defusing conflict between patients. Or perhaps they intervene in a way that tends to divert a would-be assailant’s anger away from the other patient and toward the staff, thereby reducing the rate of assaults against patients but increasing the rate of assaults against hospital personnel. But that is speculation. Alternatively, the rate of assaults against patients may have some effect on RN staffing levels (causation in the opposite direction).
Although one previous study reported nonlinear associations between staffing levels and assault rates (2), there was little evidence of such associations in this study. The BIC tends to favor more parsimonious models, but even in the best (according to the BIC) nonlinear model for each criterion variable, the quadratic staffing term was not statistically significant. This seeming discrepancy may be due to differences in the models and variables used in the two studies, or it may be attributable to this study’s use of data at the unit level versus the previous study’s use of data at the unit-month level. Measures aggregated across months are more stable, but in this study, aggregation may have “averaged out” some nuances in the monthly data.
It is worth noting that assaults against patients accounted for 25% of the injurious assaults in this study, and conflict between patients was an antecedent in an estimated 23% of incidents of violence or aggression in the meta-analysis cited previously (4). This may not be entirely coincidental. If the correspondence between conflicts among patients and assaults on patients is roughly one to one (in the sense that most assaults against patients are triggered by conflicts between patients, and most conflicts between patients triggers only assaults against patients), it would follow that assaults with other antecedents are largely directed toward hospital personnel, underscoring the importance of understanding the antecedents of assault, including patient-staff interactions.
Because the study sample comprised units from hospitals participating in the NDNQI, it was not a random or proportional sample. The findings of this study are generalizable to the extent that the staffing-violence associations observed in the sample resemble those in psychiatric units more generally. Differences among units in patient population were not controlled for, given that patient-level data were unavailable.
Conclusions
Injurious assaults against hospital personnel and against patients differed in their associations with nurse staffing levels and should be studied as separate phenomena with different causes. The results of this study confirm and qualify previous findings of a positive staffing-violence association: higher levels of staffing by non-RNs were associated with higher rates of injurious assaults, regardless of victim, whereas higher levels of staffing by RNs were associated with higher rates of injurious assaults against hospital personnel and with lower rates of injurious assaults against patients.
Clearly there is need for further research on associations between nurse staffing and patient violence. Studies that include patient-level data would allow for control of differences in patient mix, and researchers should consider studies exploiting natural variations in staffing across time. Hospitals could carry out small experiments, adjusting staffing on a randomly selected set of units and observing any change in assault rates compared with a set of control units. Such research efforts could provide insight into the direction of causation between staffing and violence, potentially with practical implications for psychiatric unit staffing.
1 : Inpatient Violence and Aggression: A Literature Review. Report From the Conflict and Containment Reduction Research Programme. London, , 2011Google Scholar
2 : Nurse staffing, RN mix, and assault rates on psychiatric units. Research in Nursing and Health 36:26–37, 2013Crossref, Medline, Google Scholar
3 : Identifying key factors associated with aggression on acute inpatient psychiatric wards. Issues in Mental Health Nursing 30:260–271, 2009Crossref, Medline, Google Scholar
4 : The antecedents of violence and aggression within psychiatric in-patient settings. Acta Psychiatrica Scandinavica 125:425–439, 2012Crossref, Medline, Google Scholar
5 : Nursing staff numbers and their relationship to conflict and containment rates on psychiatric wards—a cross sectional time series poisson regression study. International Journal of Nursing Studies 49:15–20, 2012Crossref, Medline, Google Scholar
6 : Assisted and unassisted falls: different events, different outcomes, different implications for quality of hospital care. Joint Commission Journal on Quality and Patient Safety 40:358–364, 2014Crossref, Medline, Google Scholar
7 Hedeker D: Generalized linear mixed models; in Encyclopedia of Statistics in Behavioral Science. Hoboken, NJ, Wiley, 2005. Available at www.wiley.com/legacy/wileychi/eosbs/pdfs/bsa251.pdfGoogle Scholar
8 : Negative Binomial Regression. New York, Cambridge University Press, 2007Crossref, Google Scholar
9 : The Bayesian Information Criterion: background, derivation, and applications. Wiley Interdisciplinary Reviews: Computational Statistics 4:199–203, 2012Crossref, Google Scholar
10 : Summarizing the predictive power of a generalized linear model. Statistics in Medicine 19:1771–1781, 2000Crossref, Medline, Google Scholar
11 : Violence and aggression in psychiatric units. Psychiatric Services 49:1452–1457, 1998Link, Google Scholar